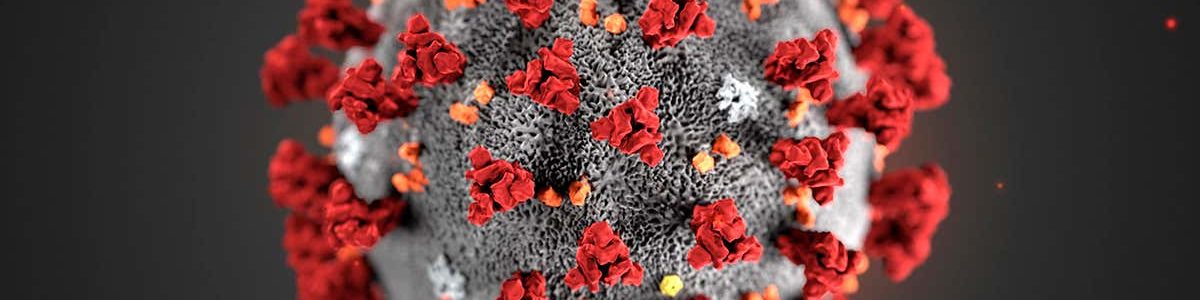
Our group will provide regular updates during the pandemic to the analysis including longer follow-up and pooled analysis with other organisations. Whether these results also apply to infection severity in the non-hospital setting or to different global populations requires further study.
Status: in-progress | First online: 29-03-2020 | Last update: 01-06-2020
Research Articles
- Using smartphones and wearable devices to monitor behavioural changes during COVID-19
arXiv:2004.14331
Shaoxiong Sun, Amos Folarin, Yatharth Ranjan, Zulqarnain Rashid, Pauline Conde, Callum Stewart, Nicholas Cummins, Faith Matcham, Gloria Dalla Costa, Letizia Leocani, Per Soelberg Sørensen, Mathias Buron, Ana Isabel Guerrero, Ana Zabalza, Brenda WJH Penninx, Femke Lamers, Sara Siddi, Josep Maria Haro, Inez Myin-Germeys, Aki Rintala, Vaibhav A. Narayan, Giancarlo Comi, Matthew Hotopf, Richard JB Dobson (on behalf of the RADAR-CNS consortium)
- ACE-inhibitors and Angiotensin-2 Receptor Blockers are not associated with severe SARS-COVID19 infection in a multi-site UK acute Hospital Trust
medRxiv 2020.04.07.20056788
Daniel M Bean, Zeljko Kraljevic, Thomas Searle, Rebecca Bendayan, Kevin O’Gallagher, Andrew Pickles, Amos Folarin, Lukasz Roguski, Kawsar Noor, Anthony Shek, Rosita Zakeri, Ajay M Shah, James TH Teo, Richard JB Dobson
- Impact of ethnicity on outcome of severe COVID-19 infection. Data from an ethnically diverse UK tertiary centre
medRxiv 2020.05.02.20078642
James T Teo, Daniel Bean, Rebecca Bendeyan, Richard Dobson,Ajay Shah
- Supplementing the National Early Warning Score (NEWS2) for anticipating early deterioration among patients with COVID-19 infection
medRxiv 2020.04.24.20078006
Ewan Carr, Rebecca Bendayan, Kevin O’Gallagher, Daniel Bean, Andrew Pickles, Daniel Stahl, Rosita Zakeri, Thomas Searle, Anthony Shek, Zeljko Kraljevic, James T Teo, Ajay Shah, Richard Dobson
- The effects of ARBs, ACEIs and statins on clinical outcomes of COVID-19 infection among nursing home residents
medRxiv 2020.05.11.20096347
Anton De Spiegeleer, Antoon Bronselaer, James T Teo, Geert Byttebier, Guy De Tre, Luc Belmans, Richard Dobson, Evelien Wynendaele, Christophe Van De Wiele, Filip Vandaele, Diemer Van Dijck, Daniel Bean, David Fedson, Bart De Spiegeleer
- Risk prediction for poor outcome and death in hospital in-patients with COVID-19: derivation in Wuhan, China and external validation in London, UK
medRxiv 2020.04.28.20082222
Huayu Zhang, Ting Shi, Xiaodong Wu, Xin Zhang, Kun Wang, Daniel Bean, Richard Dobson, James T Teo, Jiaxing Sun, Pei Zhao, Chenghong Li, Kevin Dhaliwal, Honghan Wu, Qiang Li, Bruce Guthrie
In the News
- 28 May 2020. Risk factors for BAME groups need to be understood: Experts [EasternEye]
- 25 May 2020. Ethnic minority COVID-19 patients in London are 10 years YOUNGER than white ones, study finds [Brinkwire]
- 23 May 2020. BAME people in hospital with corona ‘10yrs younger’ than others [The News International]
- 22 May 2020. BAME Covid-19 patients in London are 11 years YOUNGER than white people with the disease, study finds [The Daily Mail]
- 22 May 2020. BAME people in hospital with coronavirus ’10 years younger than white patients’ [ITV News]
- 22 May 2020. BAME people in hospital with coronavirus ’10 years younger than white patients’ [Yahoo News]
- 22 May 2020. BAME people in hospital with coronavirus ’10 years younger than white patients’ [The Irish Examiner]
- 22 May 2020. BAME COVID-19 patients 10 years younger than white counterparts, study finds [King’s College London]
ACE-inhibitors and Angiotensin-2 Receptor Blockers are not associated with severe SARS-COVID19 infection in a multi-site UK acute Hospital Trust
First online: 29-03-2020 | Last update: 12-05-2020
Authors
Daniel M Bean1,2+, Zeljko Kraljevic1, Thomas Searle1, Rebecca Bendayan1,4, Kevin O’Gallagher5,6, Andrew Pickles1, Amos Folarin1,2,3,7, Lukasz Roguski2,3,7, Kawsar Noor2,3,7, Anthony Shek8, Rosita Zakeri5,6, Ajay M Shah5,6+*, James TH Teo5,8+*, Richard JB Dobson1,2,3,4,7+*
*joint author +joint corresponding authors
Affiliations
- Department of Biostatistics and Health Informatics, Institute of Psychiatry, Psychology and Neuroscience, King’s College London, London, U.K.
- Health Data Research UK London, University College London, London, U.K.
- Institute of Health Informatics, University College London, London, U.K.
- NIHR Biomedical Research Centre at South London and Maudsley NHS Foundation Trust and King’s College London, London, U.K.
- Kings College Hospital NHS Foundation Trust, London, U.K.
- School of Cardiovascular Medicine & Sciences, King’s College London British Heart Foundation Centre of Excellence, London SE5 9NU, U.K.
- NIHR Biomedical Research Centre at University College London Hospitals NHS Foundation Trust, London.
- Dept of Clinical Neuroscience, Institute of Psychiatry, Psychology and Neuroscience, King’s College London, London
Correspondence: Prof Richard Dobson, Dept of Biostatistics and Health Informatics, Institute of Psychiatry, Psychology and Neuroscience, King’s College London, 6 De Crespigny Park, London SE5 8AF, UK (richard.j.dobson@kcl.ac.uk).
Or: Dr Dan Bean (daniel.bean@kcl.ac.uk); Dr James Teo (jamesteo@nhs.net); Prof Ajay Shah (ajay.shah@kcl.ac.uk)
Citation
Bean, Daniel, et al. “ACE-inhibitors and Angiotensin-2 Receptor Blockers are not associated with severe SARS-COVID19 infection in a multi-site UK acute Hospital Trust.”medRxiv 2020.04.07.20056788. https://doi.org/10.1101/2020.04.07.20056788
Abstract:
Aims: The SARS-Cov2 virus binds to the ACE2 receptor for cell entry. It has been suggested that ACE-inhibitors (ACEi) and Angiotensin-2 Blockers (ARB), which are commonly used in patients with hypertension or diabetes and may raise ACE2 levels, could increase the risk of severe COVID19 infection.
Methods and Results: We evaluated this hypothesis in a consecutive cohort of 1200 acute inpatients with COVID19 at two hospitals with a multi-ethnic catchment population in London (UK). The mean age was 68±17 years (57% male) and 74% of patients had at least 1 comorbidity. 415 patients (34.6%) reached the primary endpoint of death or transfer to a critical care unit for organ support within 21-days of symptom onset. 399 patients (33.3 %) were taking ACEi or ARB. Patients on ACEi/ARB were significantly older and had more comorbidities. The odds ratio (OR) for the primary endpoint in patients on ACEi and ARB, after adjustment for age, sex and co-morbidities, was 0.63 (CI 0.47-0.84, p<0.01).
Conclusions: There was no evidence for increased severity of COVID19 disease in hospitalised patients on chronic treatment with ACEi or ARB. A trend towards a beneficial effect of ACEi/ARB requires further evaluation in larger meta-analyses and randomised clinical trials.
Keywords: COVID19, angiotensin converting enzyme inhibitors, hypertension, disease outcome
Introduction
The SARS-Cov2 pandemic is a major medical and socioeconomic challenge with at least 3 million confirmed cases to date. Data on the clinical characteristics of patients who require hospital admission for COVID19 disease from China, Italy and the US consistently show that patients with cardiovascular comorbidities are over-represented and may have an increased risk of severe COVID19 disease.1–3 The reasons underlying the increased incidence of severe COVID19 infection in those with comorbidities such as hypertension, diabetes and other cardiovascular conditions are unknown.
The SARS-Cov2 virus requires the binding of its viral surface spike protein to the ACE2 receptor expressed on epithelial cells in order to be internalised and then undergo replication.4 Previous studies suggest that the expression of ACE2 may be increased by chronic treatment with ACEi or ARB.5 As such, it has been hypothesized that treatment with ACEi or ARB could increase the likelihood of SARS-Cov2 binding and entry into epithelial or other cells.6 Furthermore, it is hypothesised that such a mechanism could account for the increased incidence of severe COVID19 infection among patients with cardiovascular comorbidities, who are frequently treated with ACEi/ARB.6 Whether or not treatment with ACEi/ARB increases the risk of severe COVID19 disease is a very important question in view of the large numbers of patients potentially on these drugs, especially in western countries with older populations. The issue is controversial because ACEi/ARB may potentially be beneficial in severe lung injury by reducing activation of the renin angiotensin system (RAS).7,8 Furthermore, increased levels of ACE2 itself have been shown to be protective during severe lung injury.9,10 The potential effect of ACEi and ARB during infection with SARS-CoV-2 therefore requires urgent clarification.
We tested for association between treatment with ACEi/ARB and disease severity in a consecutive series of 1200 patients with COVID19 disease admitted to two UK hospitals, King’s College Hospital and Princess Royal University Hospital, that have been at the epicentre of the pandemic in London. We used an established and validated informatics pipeline to allow rapid evaluation of this important question during the pandemic.
Methods
This project operated under London South East Research Ethics Committee approval (reference 18/LO/2048) granted to the King’s Electronic Records Research Interface (KERRI); specific work on COVID19 research was reviewed with expert patient input on a virtual committee with Caldicott Guardian oversight.
Study Design:
The study cohort was defined as all adult inpatients testing positive for SARS-Cov2 by RT-PCR at King’s College Hospital and Princess Royal University Hospital from 1st March to 13th April 2020. Only symptomatic patients who required inpatient admission were included. Presenting symptoms included but were not limited to fever, cough, dyspnoea, myalgia, chest pain or delirium. The primary endpoint was defined as death or admission to a critical care unit for organ-support within 21 days of symptoms onset. Data were collected for a range of clinical and demographic parameters (Table 1). To ascertain chronic treatment with ACEi, ARB and other relevant medications, we captured information from clinical notes, outpatient clinic letters and inpatient medication orders. If a drug was a regular medication in the community but withheld on admission, we considered this to be on chronic treatment. The primary endpoint was manually verified by clinician review of the electronic health record.
Data Processing:
The data (demographic, emergency department letters, discharge summaries, clinical notes, radiology reports, medication orders, lab results) was retrieved and analysed in near real-time from the structured and unstructured components of the electronic health record (EHR) using a variety of well-validated natural language processing (NLP) informatics tools belonging to the CogStack ecosystem,11 namely DrugPipeline,12 MedCAT13 and MedCATTrainer.14 The CogStack NLP pipeline captures negation, synonyms, and acronyms for medical SNOMED-CT concepts as well as surrounding linguistic context using deep learning and long short-term memory networks. DrugPipeline was used to annotate medications and MedCAT produced unsupervised annotations for all SNOMED-CT concepts under parent terms Clinical Finding, Disorder, Organism, and Event with disambiguation, pre-trained on MIMIC-III.15 Further supervised training improved detection of annotations and meta-annotations such as experiencer (is the concept annotated experienced by the patient or other), negation (is the concept annotated negated or not) and temporality (is the concept annotated in the past or present ) with MedCATTrainer. Meta-annotations for hypothetical and experiencer were merged into Irrelevant meaning that any concept annotated as either hypothetical or where the experiencer was not the patient was annotated as irrelevant. Performance of the MedCAT NLP pipeline for disorders mentioned in the text was evaluated on 5617 annotations for 265 documents by a domain expert (JTHT) and F1, precision and recall recorded. Additional full case review for correct subsequent diagnosis assignment was performed by 3 clinicians (JTHT, KOG, RZ) for key comorbidities. The performance of DrugPipeline has previously been described.12 Manual review of 100 detections gave F1=0.91 for exclusion of drug allergies by DrugPipeline.
Statistical Analysis:
In order to investigate the association between ACEi/ARB and disease severity measured as critical care admission or death, we performed a series of logistic regressions. In a first step, we explored independently the association for ACEi/ARB (Baseline model). In a second step, we adjusted the model for age and sex (Model 1). Then, we additionally adjusted for hypertension (Model 2) and finally, additionally adjusted for other comorbidities, i.e. diabetes, ischemic heart disease, heart failure and chronic kidney disease (Model 3). We also explored the independent association for hypertension following the same modelling approach. In addition, we assessed the robustness to unmeasured confounders of the fully adjusted estimate of ACEi/ARB effect using the e-value approach, which are defined as the minimum strength of association on the risk-ratio scale that an unmeasured confounder would need to have with both the treatment assignment and the outcome to fully explain away a specific treatment-outcome association, conditional on the measured covariates16. Sensitivity analyses were performed i) requiring at least two detections of medication for positive exposure; ii) using only structured data on in-hospital medication orders; iii) ignoring our 21 day window for medications; iv) testing sensitivity to unmeasured confounders.
Role of the funding source:
The funders had no role in study design, data collection and analysis, decision to publish, or preparation of the manuscript.
Results
Our total cohort consisted of 1200 confirmed positive symptomatic inpatients aged 63+20 (SD) years with 52% being male (Table 1). The patients were of diverse ethnicities with over 30% from minority ethnic groups. Nearly 75% of patients had one or more comorbidities. The commonest comorbidities were hypertension (51.2%), diabetes (30.2%), chronic kidney disease (17.2%), and ischaemic heart disease or heart failure (22.2%). 15.2% of patients had a BMI greater than 30 kg/m2. A total of 399 patients (33.2%) were on chronic treatment with ACEi or ARB. 415 of the 1200 patients (34.6%) required admission to the Critical Care Unit or had died within 21 days of symptom onset. Among patients who achieved the primary endpoint (Death or Critical Care admission), the percentage who had positive mentions for various disorders derived via the NLP for medical concept annotations with F1 > 80% and more than 10 annotated mentions, as compared to those not achieving the primary endpoint, is shown in Figure 1. The performance of the NLP pipeline is shown in Figure 2. Manual validation of the presence of comorbidities was performed in a sample of 200 patients and showed excellent performance, for example a false positive rate of 1% for hypertension and 0% for diabetes.
We next compared the outcome of patients on chronic treatment with ACEi/ARB versus those not on these agents. The group on ACEi/ARB were significantly older but had a similar male/female split and a similar ethnicity profile to those not on ACEi/ARB (Table 1). The BMI was similar between groups. There was a greater proportion of patients with cardiovascular comorbidities (hypertension, diabetes, heart failure, ischaemic heart disease) and chronic kidney disease in the group on ACEi/ARB than those not taking these drugs, as would be expected. Therefore, the patients on ACEi/ARB had a higher prevalence of factors associated with worse outcome of COVID19 disease in prior studies.1–3 The ACEi/ARB group also had higher rates of treatment with beta blockers and statins than those not on ACEi/ARB, consistent with their higher rates of cardiovascular morbidities. Figure 3 shows Kaplan-Meier curves for the primary end-point in patients on ACEi/ARB and those not on these drugs.
To assess the independent effect of ACEi/ARB on primary outcome, we first performed an unadjusted logistic regression analysis. This indicated that the likelihood of a severe outcome was similar in individuals on ACEi/ARB as compared to those not on these drugs, with an Odds Ratio (OR) of 0·83 (CI 0·64-1·07) – Baseline Model, Table 2. However, after adjustments for age and sex (Model 1 in Table 2), the likelihood of severe disease was significantly lower in those on ACEi/ARBs (OR 0.70 [0.53-0.91], p<0.01). Additional adjustment for hypertension (Model 2 in Table 2) and for the other major comorbidities, diabetes, chronic kidney disease, and ischaemic heart disease/heart failure (Model 3 in Table 2), had a modest further effect. The OR for the primary outcome in Model 3 was 0.63 (0.47-0.84), p<0.01. Supplementary Table 1 shows the OR and p-values for all variables in each model. Male patients were found to have a higher likelihood of severe disease in Model 3 (OR 1·50 [CI 1·17-1·93], p=<0.01).
We also examined the independent association between hypertension and disease severity. The results showed that individuals with hypertension had a similar likelihood of suffering a severe outcome as those that without hypertension, either in unadjusted models (OR 1·25 [CI 0·98-1·59]; p=0·069) or in models adjusted for age and gender (OR 1·03 [CI 0·80-1·32]; p=0·83).
Sensitivity analyses were performed using criteria for ACEi exposure that were either more strict (requiring multiple mentions in the clinical notes or using only in-hospital medication orders as evidence) or less strict (including any mention of ACEi treatment even outside a 21 day window from onset of symptoms). In all cases, the estimates of the impact of ACEi treatment were consistent with those in Table 2. In analysis requiring at least two mentions of chronic treatment with ACEi/ARB, we found that this was significant in the uncorrected baseline model (a lower OR). We estimated an e-value of 1.818 which suggests that the estimate could be vulnerable to possible confounders not yet included.
Discussion
This study in a large consecutive cohort of 1200 patients in the UK suggests that chronic treatment with ACEi and ARB is not associated with an increase in severe outcome of COVID19 disease, defined as death or admission to a critical care unit. The hypothetical relationship between treatment with ACEi/ARB and severe COVID19 disease has been intensely debated.6-7,8 There are theoretical mechanisms whereby chronic treatment with ACEi/ARB might increase propensity to SARS-CoV2 infection as well as other mechanisms whereby treatment with these agents might be beneficial. It is a particularly important question because chronic treatment with ACEi/ARB is of proven benefit in conditions such as hypertension, diabetes, chronic kidney disease and heart failure and an unwarranted cessation of therapy in patients with these conditions as a result of the SARS-CoV2 pandemic could have serious long-term detrimental effects.
The general clinical characteristics and the rates of severe outcome of the patients in our study were broadly similar to those that have been described in recent large series from Italy and the USA.1–3 We found that patients who were on chronic treatment with ACEi/ARB had many demographic and comorbidity features that have been associated in previous studies with worse outcome in COVID-19 disease, such as an older age and a higher prevalence of hypertension, diabetes, heart failure and other morbidities1–3. Treatment with ACEi/ARB was nevertheless not associated with an increase in rates of severe outcomes, with or without adjustment for age, sex and comorbidities. Two very recent studies from China have reported on the relationship between ACEi/ARB and outcome of COVID-19 disease in hospitalised patients. In a single centre study from Wuhan in which only 115 of 1178 patients (<10%) were taking ACEi/ARB, the authors did not find any relationship between these drugs and outcome,17 the data are however limited by the low numbers on ACEi/ARB and potential confounding by other factors. A second report was a retrospective multi-centre study including 1128 patients but again had only 188 patients (16.6%) on treatment with ACEi/ARB.18 This study suggested that treatment with ACEi/ARB was associated with a lower rate of severe outcome with COVID19 infection. Our study is the first to be conducted on an ethnically mixed population in the western world and includes significant proportions of both White and minority ethnic (Black, Asian) patients. The rates of usage of ACEi/ARB in our study (33.2%) are in line with those expected in well-treated patients with comorbidities and are therefore, in principle, more applicable to patients in Europe and the Americas. Ethnicity is a very pertinent issue in this regard due to the recognised ethnicity-related differences in response to drugs affecting the RAS.19,20 Of relevance, the ethnicity profiles of the patients on ACEi/ARB in our study were similar to those not taking these drugs.
In the current study, when we adjusted for age, sex and comorbidities in logistic regression analyses, the OR for a severe outcome was significantly lower in patients on ACEi/ARB than those not on these agents. This suggestion of a favourable association of treatment with ACEi/ARB and less severe outcome in COVID19 disease would be consistent with the hypothesised beneficial effects of inhibition of RAS activation in patients with severe lung injury or Acute Respiratory Distress Syndrome (ARDS).7,8 However due to possibility of unmeasured confounds, the confirmation of a potential therapeutic benefit of treatment with ACEi/ARB in COVID19 disease would require further studies and randomised control trials.
This study used an NLP approach to perform very rapid analysis of high volume, unstructured real world clinical data. This however introduces the possibility of missing circumlocutory mentions of disease, symptoms or medications. We mitigated against this by manually validating annotations in a subset of records and also verifying drug treatments against inpatient electronic prescription data. Moreover, we performed sensitivity analyses to test the impact of different criteria to define the ACEi/ARB exposed cohort on our results, and found that the OR remained <1.0 and significant for ACEi/ARB exposure in all adjusted analyses. We therefore consider our analysis pipeline to be robust to specific details of the pipeline that are not clinically relevant. However we did find that the estimated odds ratio may be sensitive to unmeasured confounding, which suggests caution in the interpretation of any protective effect and the need for replication in a larger sample remains.
Our study has some potential limitations. Although the patients and data were prospectively collected, the analyses were retrospective. The study was conducted on two hospital sites in a single geographical, albeit ethnically mixed, locus in the UK over a relatively short follow-up period. However, the duration of follow-up is sufficient to accurately detect early severe outcomes based on the data from multiple studies during the current pandemic. We used the covariates identified as important in the previous large case series on COVID19 1–3, including age, sex and common comorbidities, to adjust our analyses. However, it is possible that other unmeasured confounders could have influenced the results. For example, the patients on chronic ACEi/ARB treatment were also more frequently treated with statins than those not on these drugs, which could suggest that their medical conditions were generally better managed. However, the ACEi/ARB group was also older and had higher rates of hypertension, diabetes and multiple morbidities, making it unlikely that these patients were physiologically healthier. Our study was performed in patients with COVID19 who required hospitalisation; the effect of chronic treatment with ACEi/ARB on less severe infection with SARS-CoV2 in the non-hospital setting requires further study. Whether the current results are applicable to other global populations, such as in Africa, will also require additional study.
In summary, the results of this study in 1200 patients show no evidence of a detrimental effect of chronic treatment with ACEi/ARB in patients presenting with severe COVID19 infection. Patients on treatment with ACEi/ARB should continue these drugs during their COVID19 illness as per current ESC Council guidelines.21
Acknowledgements
DMB is funded by a UKRI Innovation Fellowship (Health Data Research UK MR/S00310X/1).
RB is funded in part by the King’s College London UK Medical Research Council (MRC) Skills Development Fellowship programme (MR/R016372/1) and the National Institute for Health Research (NIHR) Biomedical Research Centre at South London and Maudsley NHS Foundation Trust and King’s College London (SLAM-KCL; IS-BRC-1215-20018).
AF is supported by the NIHR Biomedical Research Centre at SLAM-KCL (IS-BRC-1215-20018) and the NIHR centre at University College London Hospitals.
RJBD is supported by Health Data Research UK; the BigData@Heart Consortium, funded by the Innovative Medicines Initiative-2 Joint Undertaking (Grant No. 116074) under the European Union Horizon 2020 programme; the NIHR University College London Hospitals Biomedical Research Centre; the NIHR Biomedical Research Centre at SLAM-KCL.
KO’G is supported by an MRC Clinical Training Fellowship.
RZ is supported by a King’s Prize Fellowship.
AS is supported by a King’s Medical Research Trust studentship.
AMS is supported by the British Heart Foundation (CH/1999001/11735), the NIHR Biomedical Research Centre at Guy’s & St Thomas’ NHS Foundation Trust and King’s College London (IS-BRC-1215-20006), and the Fondation Leducq.
AP is partially supported by NIHR NF-SI-0617-10120.
This work was supported by the NIHR University College London Hospitals Biomedical Research Centre Clinical and Research Informatics Unit; NIHR Health Informatics Collaborative; the Institute of Health Informatics at University College London; and Health Data Research UK. The manuscript represents independent research part-funded by the NIHR Biomedical Research Centre at SLAM-KCL. The views expressed are those of the authors and not necessarily those of the NHS, the NIHR or the Department of Health and Social Care. The funders had no role in study design, data collection and analysis, decision to publish, or preparation of the manuscript.
We thank all the clinicians managing the patients, the patient experts of the KERRI committee, AI4VBH, Professor Irene Higginson, Professor Alastair Baker, Professor Jules Wendon, Dan Persson and Damian Lewsley for their support.
Declaration of Interests
JTHT received research support and funding from InnovateUK, Bristol-Myers-Squibb, iRhythm Technologies, and holds shares <£5,000 in Glaxo Smithkline and Biogen.
Author Contributions
JTHT, RJBD, DMB, ZK, TS, AF conceived the study design
DMB, ZK, AS, TS, JTHT, LR, KN performed data processing and software development
KOG, RZ, JTHT performed data validation
DMB, AP, RB performed statistical analysis
JTHT, DMB, ZK, TS, AF, RJBD, AMS performed critical review
JTHT, RJBD, DMB, AMS, ZK, TS, AF, DMB, AP, RB wrote the manuscript
References:
- Guan W-J, Ni Z-Y, Hu Y, Liang W-H, Ou C-Q, He J-X, Liu L, Shan H, Lei C-L, Hui DSC, Du B, Li L-J, Zeng G, Yuen K-Y, Chen R-C, Tang C-L, Wang T, Chen P-Y, Xiang J, Li S-Y, Wang J-L, Liang Z-J, Peng Y-X, Wei L, Liu Y, Hu Y-H, Peng P, Wang J-M, Liu J-Y, Chen Z, et al. Clinical Characteristics of Coronavirus Disease 2019 in China. N Engl J Med2020;382:1708–1720.
- Grasselli G, Zangrillo A, Zanella A, Antonelli M, Cabrini L, Castelli A, Cereda D, Coluccello A, Foti G, Fumagalli R, Iotti G, Latronico N, Lorini L, Merler S, Natalini G, Piatti A, Ranieri MV, Scandroglio AM, Storti E, Cecconi M, Pesenti A, COVID-19 Lombardy ICU Network. Baseline Characteristics and Outcomes of 1591 Patients Infected With SARS-CoV-2 Admitted to ICUs of the Lombardy Region, Italy. JAMA 2020; DOI:10.1001/jama.2020.5394
- Richardson S, Hirsch JS, Narasimhan M, Crawford JM, McGinn T, Davidson KW, and the Northwell COVID-19 Research Consortium, Barnaby DP, Becker LB, Chelico JD, Cohen SL, Cookingham J, Coppa K, Diefenbach MA, Dominello AJ, Duer-Hefele J, Falzon L, Gitlin J, Hajizadeh N, Harvin TG, Hirschwerk DA, Kim EJ, Kozel ZM, Marrast LM, Mogavero JN, Osorio GA, Qiu M, Zanos TP. Presenting Characteristics, Comorbidities, and Outcomes Among 5700 Patients Hospitalized With COVID-19 in the New York City Area. JAMA 2020; DOI:10.1001/jama.2020.6775
- Hoffmann M, Kleine-Weber H, Schroeder S, Krüger N, Herrler T, Erichsen S, Schiergens TS, Herrler G, Wu N-H, Nitsche A, Müller MA, Drosten C, Pöhlmann S. SARS-CoV-2 Cell Entry Depends on ACE2 and TMPRSS2 and Is Blocked by a Clinically Proven Protease Inhibitor. Cell 2020; DOI:10.1016/j.cell.2020.02.052
- Vuille-dit-Bille RN, Camargo SM, Emmenegger L, Sasse T, Kummer E, Jando J, Hamie QM, Meier CF, Hunziker S, Forras-Kaufmann Z, Kuyumcu S, Fox M, Schwizer W, Fried M, Lindenmeyer M, Götze O, Verrey F. Human intestine luminal ACE2 and amino acid transporter expression increased by ACE-inhibitors. Amino Acids 2015;47:693–705.
- Zheng Y-Y, Ma Y-T, Zhang J-Y, Xie X. COVID-19 and the cardiovascular system. Nat Rev Cardiol 2020;17:259–260.
- Vaduganathan M, Vardeny O, Michel T, McMurray JJV, Pfeffer MA, Solomon SD. Renin-Angiotensin-Aldosterone System Inhibitors in Patients with Covid-19. N Engl J Med 2020;382:1653–1659.
- Kuster GM, Pfister O, Burkard T, Zhou Q, Twerenbold R, Haaf P, Widmer AF, Osswald S. SARS-CoV2: should inhibitors of the renin–angiotensin system be withdrawn in patients with COVID-19? Eur Heart J 2020; DOI:10.1093/eurheartj/ehaa235
- Imai Y, Kuba K, Rao S, Huan Y, Guo F, Guan B, Yang P, Sarao R, Wada T, Leong-Poi H, Crackower MA, Fukamizu A, Hui C-C, Hein L, Uhlig S, Slutsky AS, Jiang C, Penninger JM. Angiotensin-converting enzyme 2 protects from severe acute lung failure. Nature 2005;436:112–116.
- Kuba K, Imai Y, Rao S, Gao H, Guo F, Guan B, Huan Y, Yang P, Zhang Y, Deng W, Bao L, Zhang B, Liu G, Wang Z, Chappell M, Liu Y, Zheng D, Leibbrandt A, Wada T, Slutsky AS, Liu D, Qin C, Jiang C, Penninger JM. A crucial role of angiotensin converting enzyme 2 (ACE2) in SARS coronavirus–induced lung injury. Nat Med; 2005;11:875–879.
- Jackson R, Kartoglu I, Stringer C, Gorrell G, Roberts A, Song X, Wu H, Agrawal A, Lui K, Groza T, Lewsley D, Northwood D, Folarin A, Stewart R, Dobson R. CogStack – experiences of deploying integrated information retrieval and extraction services in a large National Health Service Foundation Trust hospital. BMC Med Inform Decis Mak 2018;18:47.
- Bean DM, Teo J, Wu H, Oliveira R, Patel R, Bendayan R, Shah AM, Dobson RJB, Scott PA. Semantic computational analysis of anticoagulation use in atrial fibrillation from real world data. PLoS One 2019;14:e0225625.
- Kraljevic Z, Bean D, Mascio A, Roguski L, Folarin A, Roberts A, Bendayan R, Dobson R. MedCAT — Medical Concept Annotation Tool. arXiv [cs.CL]. 2019.
- Searle T, Kraljevic Z, Bendayan R, Bean D, Dobson R. MedCATTrainer: A Biomedical Free Text Annotation Interface with Active Learning and Research Use Case Specific Customisation. Proceedings of the 2019 Conference on Empirical Methods in Natural Language Processing and the 9th International Joint Conference on Natural Language Processing (EMNLP-IJCNLP): System Demonstrations Stroudsburg, PA, USA: Association for Computational Linguistics; 2019. p. 139–144.
- Johnson AEW, Pollard TJ, Shen L, Lehman L-WH, Feng M, Ghassemi M, Moody B, Szolovits P, Celi LA, Mark RG. MIMIC-III, a freely accessible critical care database. Sci Data 2016;3:160035.
- Linden A, Mathur MB, VanderWeele TJ. Conducting sensitivity analysis for unmeasured confounding in observational studies using E-values: The evalue package. Stata J 2020;20:162–175.
- Li J, Wang X, Chen J, Zhang H, Deng A. Association of Renin-Angiotensin System Inhibitors With Severity or Risk of Death in Patients With Hypertension Hospitalized for Coronavirus Disease 2019 (COVID-19) Infection in Wuhan, China. JAMA Cardiol 2020;Apr 23. doi: 10.1001/jamacardio.2020.1624.
- Zhang P, Zhu L, Cai J, Lei F, Qin J-J, Xie J, Liu Y-M, Zhao Y-C, Huang X, Lin L, Xia M, Chen M-M, Cheng X, Zhang X, Guo D, Peng Y, Ji Y-X, Chen J, She Z-G, Wang Y, Xu Q, Tan R, Wang H, Lin J, Luo P, Fu S, Cai H, Ye P, Xiao B, Mao W, et al. Association of Inpatient Use of Angiotensin Converting Enzyme Inhibitors and Angiotensin II Receptor Blockers with Mortality Among Patients With Hypertension Hospitalized With COVID-19. Circ Res 2020; DOI: 10.1161/CIRCRESAHA.120.317134
- Julius S, Alderman MH, Beevers G, Dahlöf B, Devereux RB, Douglas JG, Edelman JM, Harris KE, Kjeldsen SE, Nesbitt S, Randall OS, Wright JT Jr. Cardiovascular risk reduction in hypertensive black patients with left ventricular hypertrophy: the LIFE study. J Am Coll Cardiol 2004;43:1047–1055.
- Taylor AL, Wright JT Jr. Should ethnicity serve as the basis for clinical trial design? Importance of race/ethnicity in clinical trials: lessons from the African-American Heart Failure Trial (A-HeFT), the African-American Study of Kidney Disease and Hypertension (AASK), and the Antihypertensive and Lipid-Lowering Treatment to Prevent Heart Attack Trial (ALLHAT). Circulation 2005;112:3654–3660.
- Simone GD. Position Statement of the ESC Council on Hypertension on ACE-Inhibitors and Angiotensin Receptor Blockers. 2020. https://www.escardio.org/Councils/Council-on-Hypertension-(CHT)/News/position-statement-of-the-esc-council-on-hypertension-on-ace-inhibitors-and-ang (28 March 2020)
Figure Legends
Figure 1. Distribution of disorders between patients achieving the primary outcome (Death or Critical Care admission) and those not achieving it by 21 days after symptom onset. The percentage of patients that have a positive mention of a disorder in each of the two groups is shown. All diseases were extracted from free-text using Cogstack and MedCAT. Only medical concept annotations with F1 > 80% and more than 10 annotated samples are shown. Disease names that start “Any: ” are aggregate concepts for multiple specific conditions that are used in our analysis.
Figure 2. Performance of the CogStack and MedCAT NLP pipeline in detecting disease mentions within the electronic health record text. Precision (P), Recall (R) and F1 (harmonic mean of precision and recall). Only medical concept annotations with F1 > 80% and more than 10 annotated samples are shown. Disease names that start “Any: ” are aggregate concepts for multiple specific conditions that are used in our analysis.
Figure 3. Kaplan-Meier Curves for the primary endpoint in COVID19 patients on chronic treatment with ACE-inhibitors (ACEi) or Angiotensin-II Receptor Blockers (ARB) (blue line) versus those not on these drugs (orange line). The unadjusted Odds Ratio (OR) for the primary endpoint for those on ACEi/ARB was 0.83 (p =0.16); the adjusted OR was 0.63 (p<0.01).
Tables
Table 1. Characteristics of the 1200 patients positive for COVID19 at Princess Royal University Hospital and King’s College Hospital, London, UK.
All Patients | On ACEi or ARB | Not on ACEi or ARB | p-value | ||
---|---|---|---|---|---|
N | 1200 | 399 | 801 | ||
Age (SD), years | 67.96 (17.07) | 73.02 (13.46) | 65.45 (18.1) | <0.001 | |
Sex | Male | 686 (57.2%) | 231 (57.9%) | 455 (56.8%) | n.s. |
Female | 514 (42.8%) | 168 (42.1%) | 346 (43.2%) | n.s. | |
Ethnicity | Caucasian | 512 (42.7%) | 170 (42.6%) | 342 (42.7%) | n.s. |
Black | 310 (25.8%) | 105 (26.3%) | 205 (25.6%) | n.s. | |
Asian | 58 (4.8%) | 21 (5.3%) | 37 (4.6%) | n.s. | |
Unknown/ Mixed/Other | 320 (26.7%) | 103 (25.8%) | 217 (27.1%) | n.s. | |
Comorbidity | HTN | 645 (53.8%) | 339 (85.0%) | 306 (38.2%) | <0.001 |
Diabetes | 418 (34.8%) | 215 (53.9%) | 203 (25.3%) | <0.001 | |
HF | 107 (8.9%) | 65 (16.3%) | 42 (5.2%) | <0.001 | |
IHD | 160 (13.3%) | 83 (20.8%) | 77 (9.6%) | <0.001 | |
COPD | 121 (10.1%) | 42 (10.5%) | 79 (9.9%) | n.s. | |
Asthma | 169 (14.1%) | 58 (14.5%) | 111 (13.9%) | n.s. | |
CKD | 206 (17.2%) | 108 (27.1%) | 98 (12.2%) | <0.001 | |
Previous Stroke/TIA | 235 (19.6%) | 112 (28.1%) | 123 (15.4%) | <0.001 | |
BMI (SD), kg/m2 | 26.3 (8.7) | 27.0 (8.5) | 25.8 (8.4) | n.s. | |
BMI>=30 | 182 (15.2%) | 69 (17.3%) | 113 (14.1%) | n.s. | |
Number of comorbidities | 0 | 310 (25.8%) | 19 (4.8%) | 291 (36.3%) | <0.001 |
1 | 283 (23.6%) | 73 (18.3%) | 210 (26.2%) | 0.08 | |
>1 | 607 (50.6%) | 307 (76.9%) | 300 (37.5%) | <0.001 | |
Drugs | ACEi | 260 (21.7%) | 260 (65.2%) | 0 (0.0%) | |
ARB | 147 (12.2%) | 147 (36.8%) | 0 (0.0%) | ||
Statin | 472 (39.3%) | 268 (67.2%) | 204 (25.5%) | <0.001 | |
Beta-blocker | 337 (28.1%) | 184 (46.1%) | 153 (19.1%) | <0.001 | |
Vital Signs | Systolic BP (SD), mmHg | 124 (27) | 126 (28) | 123 (26) | 0.17 |
Diastolic BP (SD), mmHg | 71 (18) | 71 (18) | 71 (18) | n.s. | |
Primary endpoint by 21 days | Death or Critical Care admission | 415 (34.6%) | 127 (31.8%) | 288 (36.0%) | n.s. |
Death | 288 (24.0%) | 106 (26.6%) | 182 (22.7%) | n.s. | |
Critical Care admission and Alive | 127 (10.6%) | 21 (5.3%) | 106 (13.2%) | <0.01 |
All variables were complete and shown as N (% of column) except age which is mean (SD). ACEi = Angiotensin converting enzyme inhibitor; ARB = Angiotensin-2 Receptor Blocker.; HTN = hypertension; HF = heart failure; IHD = ischemic heart disease; COPD = chronic obstructive pulmonary disease; CKD = chronic kidney disease; TIA = transient ischaemic attack; BMI = body mass index. Data was available on all patients except for ethnicity (925), systolic blood pressure (1120), diastolic blood pressure (1120), BMI (621). P-value comparing the group on ACEi /ARB vs. Not On ACEi/ARB with Bonferroni correction for multiple testing. Continuous variables compared with a t-test, binary variables compared with a Chi-squared test.
Table 2. Summary of Odds Ratios for ACE inhibitor and/or ARB drug use and the primary endpoint.
Model | Adjustments | OR (95% CI) ACEi/ARB vs neither drug | p |
---|---|---|---|
Baseline | - | 0.83 (0.64-1.07) | 0.16 |
Model 1 | Age, sex | 0.70 (0.53-0.91) | <0.01 |
Model 2 | Age, sex, hypertension | 0.64 (0.48-0.86) | <0.01 |
Model 3 | Age, sex, hypertension, diabetes mellitus, chronic kidney disease, ischaemic heart disease, heart failure | 0.63 (0.47-0.84) | <0.01 |
Odds ratios (OR) and p-values calculated from logistic regressions. ACEi = Angiotensin converting enzyme inhibitor; ARB = Angiotensin-2 Receptor Blocker.
Figure 1. The percentage of patients that have a positive mention of a disorder in each of the two groups (Dead or Critical Care, Other).
Dead or Critical Care – patients that had died or were admitted to the Critical Care Unit; and Other – patients that were alive and had not been admitted to the Critical Care by day 21. All diseases were extracted from free-text using Cogstack and MedCAT. Only medical concept annotations with F1 > 80% and more than 10 annotated samples are shown. Disease names that start “Any: ” are aggregate concepts for multiple specific conditions that are used in our analysis.
Figure 2. Performance of the CogStack and MedCAT NLP pipeline in detecting disease mentions within the electronic health record text.
Precision (P), Recall (R) and F1 (harmonic mean of precision and recall). Only medical concept annotations with F1 > 80% and more than 10 annotated samples are shown. Disease names that start “Any: ” are aggregate concepts for multiple specific conditions that are used in our analysis.
Figure 3. Kaplan-Meier Curves for the primary endpoint in COVID19 patients on chronic treatment with ACE-inhibitors (ACEi) or Angiotensin-II Receptor Blockers (ARB) (blue line) versus those not on these drugs (orange line).
The unadjusted Odds Ratio (OR) for the primary endpoint for those on ACEi/ARB was 0.83 (p =0.16); the adjusted OR was 0.63 (p<0.01).
Supplementary files
Supplementary Table 1. Odds ratios and p-values for all variables and primary endpoint.
Model | Variable | OR (95% CI) | P-value |
---|---|---|---|
Baseline | On ACEi or ARB | 0.83 (0.64-1.07) | 0.16 |
Model 1 | On ACEi or ARB | 0.70 (0.53-0.91) | <0.01 |
Age (per 10 years) | 1.26 (1.17-1.36) | <0.01 | |
Male | 1.51 (1.18-1.93) | <0.01 | |
Model 2 | On ACEi or ARB | 0.64 (0.48-0.86) | <0.01 |
Age (per 10 years) | 1.25 (1.16-1.35) | <0.01 | |
Male | 1.51 (1.18-1.94) | <0.01 | |
HTN | 1.22 (0.92-1.60) | 0.16 | |
Model 3 | On ACEi or ARB | 0.63 (0.47-0.84) | <0.01 |
Age (per 10 years) | 1.24 (1.14-1.34) | <0.01 | |
Male | 1.50 (1.17-1.93) | <0.01 | |
HTN | 1.15 (0.86-1.55) | 0.34 | |
Diabetes | 1.07 (0.81-1.40) | 0.64 | |
HF or IHD | 0.95 (0.68-1.31) | 0.73 | |
CKD | 1.33 (0.95-1.86) | 0.09 | |
Hypertension unadjusted | HTN | 1.25 (0.98-1.59) | 0.069 |
Hypertension adjusted | Age (per 10 years) | 1.24 (1.15-1.33) | <0.01 |
Male | 1.50 (1.17-1.92) | <0.01 | |
HTN | 1.03 (0.80-1.32) | 0.83 |
Odds ratios and p-values calculated from logistic regressions. ACEi = Angiotensin converting enzyme inhibitor. OR = Odds ratio. ARB = Angiotensin Receptor Blocker. HTN = hypertension; HF = heart failure; IHD = ischaemic heart disease; CKD = chronic kidney disease.
Impact of ethnicity on outcome of severe COVID-19 infection. Data from an ethnically diverse UK tertiary centre
First online: 13-05-2020 | Last update: 21-05-2020
Authors
James T Teo1,2,*, Daniel Bean3,4,*, Rebecca Bendeyan3,5, Richard Dobson3,4,5#, Ajay Shah1,6#
*Joint First Authors
#Joint corresponding authors: Ajay M Shah (ajay.shah@kcl.ac.uk); Richard JB Dobson (richard.j.dobson@kcl.ac.uk)
Affiliations
- King’s College Hospital NHS Foundation Trust, London, U.K.
- Department of Clinical Neuroscience, Institute of Psychiatry, Psychology and Neuroscience, King’s College London, London, U.K.
- Department of Biostatistics and Health Informatics, Institute of Psychiatry, Psychology and Neuroscience, King’s College London, London, U.K.
- Health Data Research UK London, Institute of Health Informatics, University College London, London, U.K.
- NIHR Biomedical Research Centre at South London and Maudsley NHS Foundation Trust and King’s College London, London, U.K.
- School of Cardiovascular Medicine & Sciences, King’s College London British Heart Foundation Centre of Excellence, London, U.K.
Citation
Teo, James, et al. “Impact of ethnicity on outcome of severe COVID-19 infection. Data from an ethnically diverse UK tertiary centre” medRxiv 2020.05.02.20078642. https://doi.org/10.1101/2020.05.02.20078642
Abstract
During the current COVID-19 pandemic, it has been suggested that BAME background patients may be disproportionately affected compared to White but few detailed data are available. We took advantage of near real-time hospital data access and analysis pipelines to look at the impact of ethnicity in 1200 consecutive patients admitted between 1st March 2020 and 12th May 2020 to King’s College Hospital NHS Trust in London (UK).
Our key findings are firstly that BAME patients are significantly younger and have different co-morbidity profiles than White individuals. Secondly, there is no significant independent effect of ethnicity on severe outcomes (death or ITU admission) within 14-days of symptom onset, after adjustment for age, sex and comorbidities.
Introduction
As the Covid-19 pandemic extends from ethnically homogeneous countries in East Asia to multicultural populations in Europe and North America, anecdotal reports suggest a higher disease burden in Black, Asian and Minority Ethnic (BAME) groups1. Early UK audit data on COVID-19 indicate a higher proportion of BAME patients on critical care units than previous years but do not establish whether disease outcome is worse than in White patients or the factors underlying any disparity2.
Methods
This project operated under London South East Research Ethics Committee approval (reference 18/LO/2048) granted to the King’s Electronic Records Research Interface (KERRI). Specific work on COVID19 research was reviewed with expert patient input on a virtual committee with Caldicott Guardian oversight.
We evaluated the effect of ethnicity on disease outcomes in a consecutive cohort of 1200 patients admitted with COVID-19 disease to King’s College Hospital NHS Foundation Trust from 1st March 2020 to 12th May 2020. This multi-site tertiary centre in South East London serves an ethnically diverse population: approximately 30% of residents identify as BAME in the immediate vicinity of the largest site (boroughs of Southwark and Lambeth) and ~12% near the Bromley site3.
We used an informatics pipeline previously described for evaluating ACE-inhibitor risk in patients with COVID-19 disease4. The primary endpoint was death (WHO-COVID-19 Outcomes Scale 8) or admission to critical care (WHO-COVID-19 Outcomes Scale 6-7) by 14 days after symptom onset. Self-assigned ethnicity is reported according to UK census categories.
Results
The distribution of self-identified ethnicity among patients is shown in Table 1. 30.7% of patients were BAME. BAME patients (84% Black) had a significantly higher prevalence of diabetes and hypertension but were significantly younger than White patients (Table 2, Figure 1). The prevalence of ischaemic heart disease (IHD) and heart failure tended to be lower in BAME compared to White patients. The incidence of the primary end-point by 21 days after symptom onset and Kaplan-Meier curves were similar between White and BAME patients (Figure 2).
We tested whether BAME ethnicity was independently associated with the incidence of the primary outcome but did not find a significant statistical association in a series of multivariate logistic regression models adjusted for age, sex and comorbidities (hypertension, diabetes, ischaemic heart disease/ heart failure) – Table 3. Increasing age and male sex were associated with an increased likelihood of the primary outcome.
This study in a series of 1200 patients hospitalised with COVID-19 disease in London (United Kingdom) indicates large ethnicity-related differences in age and comorbidities. Patients of BAME origin who require admission for COVID-19 are significantly younger than White patients from the same geographical area and have a significant higher burden of diabetes and hypertension. We did not identify an independent impact of ethnicity on the severity of in-hospital outcome after admission. Our BAME patients were predominantly Black and the results may not apply to other ethnicities. Further studies are required to establish the reasons underlying the ethnicity-related differences in number and profile of patients who require hospitalisation for COVID-19 disease.
References:
- Croxford R. Ethnic minorities ‘a third’ of Covid-19 patients. BBC. 2020; published online April 12. https://www.bbc.com/news/uk-52255863 (accessed April 17, 2020).
- Intensive Care National Audit & Research Centre (ICNARC). Report on 3883 patients critically ill with COVID-19; published online April 4 2020. https://www.icnarc.org/DataServices/Attachments/Download/c31dd38d-d77b-ea11-9124-00505601089b (accessed April 17, 2020)
- Public Health England (PHE). Public Health Profiles. https://fingertips.phe.org.uk/search/ethnicity#page/0/gid/1/pat/6/par/E12000007/ati/101/are/E09000022/cid/4/page-options/ovw-tdo-0 (accessed April 17, 2020).
- Bean D, Kraljevic Z, Searle T, et al. Treatment with ACE-inhibitors is associated with less severe disease with SARS-Covid-19 infection in a multi-site UK acute Hospital Trust. medRxiv 2020; :2020.04.07.20056788.
- Firth D. Bias reduction of maximum likelihood estimates. Biometrika 1993; 80: 27–38.
Acknowledgements:
DMB is funded by a UKRI Innovation Fellowship as part of Health Data Research UK MR/S00310X/1 (https://www.hdruk.ac.uk).
RB is funded in part by grant MR/R016372/1 for the King’s College London MRC Skills Development Fellowship programme funded by the UK Medical Research Council (MRC, https://mrc.ukri.org) and by grant IS-BRC-1215-20018 for the National Institute for Health Research (NIHR, https://www.nihr.ac.uk) Biomedical Research Centre at South London and Maudsley NHS Foundation Trust and King’s College London.
RJBD is supported by Health Data Research UK; The BigData@Heart Consortium, European Union’s Horizon 2020 grant agreement No. 116074; The National Institute for Health Research (NIHR) University College London Hospitals Biomedical Research Centre and the NIHR Biomedical Research Centre at South London and Maudsley NHS Foundation Trust and King’s College London.
AMS is supported by the British Heart Foundation (CH/1999001/11735), the NIHR Biomedical Research Centre at Guy’s & St Thomas’ NHS Foundation Trust and King’s College London (IS-BRC-1215-20006), and the Fondation Leducq.
The views expressed are those of the author(s) and not necessarily those of the NHS, the NIHR or the Department of Health and Social Care.
We thank all the clinicians managing the patients, the patient experts of the KERRI committee, Professor Irene Higginson, Professor Alastair Baker and Professor Jules Wendon for their support.
Declaration of Interests:
JTHT received research support and funding from InnovateUK, Bristol-Myers-Squibb, iRhythm Technologies, and holds shares <£5,000 in Glaxo Smithkline and Biogen.
Figure 1. Key demographics by Ethnicity.
a) Rate of male sex, hypertension, diabetes, ischaemic heart disease or heart failure and primary endpoint. b) Distribution of age in years. In both, blue = BAME ethnicity, orange = White ethnicity. BAME = Black, Asian and Minority Ethnic, IHD = ischaemic heart disease, HF = heart failure. Asterisks indicate significance; * = p < 0.05, ** = p < 0.01, *** = p < 0.001, all others not significant (p ≥ 0.05).
Table 1. Clinical characteristics of study cohort.
Overall | BAME Ethnicity | Black Ethnicity | White Ethnicity | Asian Ethnicity | Unknown/ Mixed/Other Ethnicity | |
---|---|---|---|---|---|---|
N (%) | 1200 | 368 (30.7) | 310 (25.8) | 512 (42.7) | 58 (4.8) | 320 (26.7) |
Age | 68.0 (17.1) | 62.9 (16.4) | 63.0 (16.1) | 73.7 (15.6) | 62.3 (18.2) | 64.7 (17.4) |
Male | 686 (57.2%) | 212 (57.6%) | 172 (-55.5%) | 291 (56.8%) | 40 (69.0%) | 183 (57.2%) |
Hypertension | 645 (53.8%) | 233 (63.3%) | 201 (-64.8%) | 246 (48.0%) | 32 (55.2%) | 166 (51.9%) |
Diabetes mellitus | 418 (34.8%) | 179 (48.6%) | 152 (-49.0%) | 126 (24.6%) | 27 (46.6%) | 113 (35.3%) |
Ischaemic heart disease or heart failure | 224 (18.7%) | 54 (14.7%) | 43 (13.9%) | 109 (21.3%) | 11 (19.0%) | 61 (19.1%) |
Primary Endpoint (Death or Critical Care) | 415 (34.6%) | 123 (33.4%) | 94 (30.3%) | 185 (36.1%) | 29 (50.0%) | 107 (33.4%) |
Death | 288 (24.0%) | 68 (18.5%) | 53 (17.1%) | 151 (29.5%) | 15 (25.9%) | 69 (21.6%) |
Critical Care | 168 (14.0%) | 65 (17.7%) | 49 (15.8%) | 51 (10.0%) | 16 (27.6%) | 52 (16.2%) |
The “Unknown/Mixed/Other Ethnicity” group contains any single ethnicity that is not White or BAME, any mixed ethnicity, and any missing / not stated ethnicity. BAME = Black, Asian and Minority Ethnic. All values are N (%) except age which is mean (s.d.). All percentages are relative to column total except for the total N row, which shows percent of cohort.
Table 2. Statistical tests for differences in patient characteristics.
Variable | Test | p | p (corrected) |
---|---|---|---|
Age | t-test | <0.001 | <0.001 |
Male | Chi2 | 0.87 | 1 |
Hypertension | Chi2 | <0.001 | <0.001 |
Diabetes | Chi2 | <0.001 | <0.001 |
Ischaemic heart disease or heart failure | Chi2 | 0.016 | 0.097 |
Endpoint Status | Chi2 | 0.45 | 1 |
All tests compare BAME ethnicity vs White ethnicity. Continuous variables are compared using a t-test, count variables are compared using a Chi-squared test. P (corrected) is the p-value after Bonferroni correction for multiple comparisons.
Table 3. Summary of odds ratios in statistical models.
Variable | OR (95% CI) | P-value | Model |
---|---|---|---|
BAME Ethnicity | 0.89 (0.67-1.18) | 0.41 | Model 1 |
BAME Ethnicity | 1.09 (0.81-1.47) | 0.58 | Model 2 |
Age per 10 years | 1.23 (1.12-1.34) | <0.001 | |
Male | 1.49 (1.12-1.99) | <0.01 | |
BAME Ethnicity | 1.11 (0.80-1.52) | 0.53 | Model 3 |
Age per 10 years | 1.25 (1.14-1.38) | <0.001 | |
Male | 1.50 (1.12-2.00) | <0.01 | |
Hypertension | 0.91 (0.66-1.24) | 0.54 | |
Diabetes | 1.02 (0.74-1.40) | 0.91 | |
Ischaemic heart disease or heart failure | 0.76 (0.52-1.10) | 0.15 |
Model 1 is univariate, Models 2 and 3 are multivariate. Odds ratios and p-values calculated from logistic regressions applying Firth’s correction5. BAME = Black, Asian and Minority Ethnic Groups.
Supplementing the National Early Warning Score (NEWS2) for anticipating early deterioration among patients with COVID-19 infection
First online: 29-04-2020 | Last update: 03-05-2020
Authors
Ewan Carr*1, Rebecca Bendayan*1,4, Kevin O’Gallagher5,6, Daniel Bean1,2, Andrew Pickles1,4, Daniel Stahl1, Rosita Zakeri5,6, Thomas Searle1,4, Anthony Shek8, Zeljko Kraljevic1, James T. Teo5,8, Ajay M. Shah5,6, Richard JB Dobson1,2,3,4,7
*joint author +corresponding author: Dr Ewan Carr (ewan.carr@kcl.ac.uk), Department of Biostatistics and Health Informatics, Institute of Psychiatry, Psychology & Neuroscience (IoPPN), 16 De Crespigny Park, London, SE5 8AF.
Affiliations
- Department of Biostatistics and Health Informatics, Institute of Psychiatry, Psychology and Neuroscience, King’s College London, London, U.K.
- Health Data Research UK London, University College London, London, U.K.
- Institute of Health Informatics, University College London, London, U.K.
- NIHR Biomedical Research Centre at South London and Maudsley NHS Foundation Trust and King’s College London, London, U.K.
- King’s College Hospital NHS Foundation Trust, London, U.K.
- School of Cardiovascular Medicine & Sciences, King’s College London British Heart Foundation Centre of Excellence, London, SE5 9NU, U.K.
- NIHR Biomedical Research Centre at University College London Hospitals NHS Foundation Trust, London, U.K.
- Dept of Clinical Neuroscience, Institute of Psychiatry, Psychology and Neuroscience, King’s College London, London, U.K.
Citation
Carr, Ewan, et al. “Supplementing the National Early Warning Score (NEWS2) for anticipating early deterioration among patients with COVID-19 infection.” medRxiv 2020.04.24.20078006. https://doi.org/10.1101/2020.04.24.20078006
Abstract
Importance:
An early minimally symptomatic phase is often followed by deterioration in patients with COVID-19 infection. This study shows that the addition of age and a minimal set of common blood tests taken in patients on admission to hospital significantly improves the National Early Warning Score (NEWS2) for risk-stratification of severe COVID disease.
Objective:
To supplement the NEWS2 score with a small number of easily obtained additional demographic, physiological and blood variables indicative of severity of COVID-19 infection.
Design:
Retrospective observational cohort with internal and temporal held-out external validation.
Setting:
Acute secondary care.
Participants:
708 patients admitted to an acute multi-site UK NHS hospital with confirmed COVID-19 disease from 1st March to 5th April 2020.
Intervention:
Not applicable
Main outcome and measures:
The primary outcome was patient status at 14 days after symptom onset categorised as severe disease (WHO-COVID-19 Outcomes Scales 6-8: i.e. transferred to intensive care unit or death). 218 of the 708 patients reached the primary end point. A range of physiological and blood biomarkers were assessed for their association with the primary outcome. Adjustments included age, gender, ethnicity and comorbidities (hypertension, diabetes, heart, respiratory and kidney diseases).
Results:
NEWS2 total score was a weak predictor for severity of COVID-19 infection at 14 days (internally validated AUC = 0.628). The addition of age and common blood tests (CRP, neutrophil count, estimated GFR and albumin) provided substantial improvements to a risk stratification model but performance was still only moderate (AUC = 0.75). Common comorbidities hypertension, diabetes, heart, respiratory and kidney diseases have minor additional predictive value.
Conclusions and relevance:
Adding age and a minimal set of common blood parameters to NEWS2 improves the risk stratification of patients likely to develop severe COVID-19 outcomes. The addition of a few common parameters is likely to be much easier to implement in a short time-scale than a novel risk-scoring system.
Introduction
While approximately 80% of individuals with COVID-19 infection have mild or no symptoms1 some develop severe COVID-19 disease requiring hospital admission. As of 23rd April 2020, there have been > 2.5 million confirmed cases worldwide2. Within the subset of those requiring hospitalisation, early identification of those who deteriorate and require transfer to an intensive care unit (ICU) for organ support or may die is invaluable12.
Currently available risk scores for deterioration of acutely ill patients include (1) widely-used generic ward-based risk indices such as the National Early Warning Score (NEWS2)3 or modified sequential organ failure assessment (mSOFA)4 ; and (2) the pneumonia-specific risk index, CURB-655 which usefully capture a combination of physiological observations with limited blood markers and comorbidities. The NEWS2 is a summary score of six physiological parameters or ‘vital signs’ (respiratory rate, oxygen saturation, systolic blood pressure, heart rate, level of consciousness, temperature and supplemental oxygen dependency), used to identify patients at risk of early clinical deterioration in the UK NHS hospitals6,7. The physiological parameters assessed in the NEWS2 score – particularly patient temperature, oxygen saturations and the supplemental oxygen dependency – have been associated with COVID-19 outcomes1; however, little is known about their predictive value for the severity of COVID-19 disease. Additionally, a number of COVID-19-specific risk indices are being developed8–10 as well as unvalidated online calculators11 but generalisability is not yet known10. A Chinese study has suggested a modified version of NEWS2 with addition of age only12 but without any data on performance. With near universal usage of NEWS2 in UK NHS Trusts since March 201913, minor adaptati onto NEWS2 would be relatively easy to implement.
As the SARS-Cov2 pandemic has progressed, evidence has emerged regarding potentially useful blood biomarkers1,14–17. Although most of these early reports contain data from small numbers of patients, a number of markers have been found to be associated with severity. These include neutrophilia and lymphopenia, particularly in older adults9,16,18,19, neutrophil-to-lymphocyteratio20, raised C-Reactive Protein (CRP) and lymphocyte-to-CRP ratio20, markers of liver and cardiac injury such as alanine aminotransferase (ALT), aspartate aminotransferase (AST) and cardiac troponin21 and elevated D-dimers, ferritin and fibrinogen 2,5,7. Furthermore, plasma levels of cytokines such as IL-6 have been found to be higher in COVID-19 patients compared to controls1.
Our aim is to understand the performance of NEWS2 and identify a supplemental combination of simple clinical and blood biomarkers routinely measured in hospitals to supplement the NEWS2 score to improve prediction of a severe disease outcome at 14 days from symptom onset. To reach this aim, our specific objectives were:
- To explore independent associations of routinely measured physiological and blood parameters (including NEWS2 parameters) at or near hospital admission with disease severity (i.e., ICU admission or death), adjusting for socio-demographics and comorbidities.
- To examine which minimal combination of these potential determinants of disease severity (physiological and blood parameters, sociodemographics and comorbidities) are the best predictors of disease severity at 14 days since symptom onset; and
- To compare the predictive value of the resulting model with a model based on the NEWS2 total score alone.
Methods
Patients
The study cohort was defined as all adult inpatients testing positive for SARS-Cov2 by reverse transcription polymerase chain reaction (RT-PCR) between 1st March to 5 th April 2020 at a multi-site acute NHS hospital in South East London (UK). The catchment area of King’s College Hospital NHS Foundation Trust includes the most severely affected part of the UK during the current pandemic. All patients included in the study had symptoms consistent with COVID-19 disease (e.g. cough, fever, dyspnoea, myalgia, delirium). We excluded subjects who were seen in the emergency department but not admitted. For purposes of temporal external validation, detailed below, patients were split into training and temporal external validation samples, with those tested positive before 31st March 2020 assigned to training, and those tested positive on/after 31st March 2020 assigned to validation.
This project operated under London South East Research Ethics Committee (reference 18/LO/2048) approval granted to the King’s Electronic Records Research Interface (KERRI); specific work on COVID-19 research was reviewed with expert patient input on a virtual committee with Caldicott Guardian oversight.
Data Processing
The data (demographics, emergency department letters, discharge summaries, clinical notes, lab results, vital signs) were retrieved and analyzed in near real-time from the structured and unstructured components of the electronic health record (EHR) using a variety of natural language processing (NLP) informatics tools belonging to the CogStack ecosystem 22 , namely MedCAT 23 and MedCATTrainer 24 . The CogStack NLP pipeline captures negation, synonyms, and acronyms for medical SNOMED-CT concepts as well as surrounding linguistic context using deep learning and long short-term memory networks. MedCAT produces unsupervised annotations for all SNOMED-CT concepts under parent terms Clinical Finding, Disorder, Organism, and Event with disambiguation, pre-trained on MIMIC-III25 . The annotated SNOMED-CT terms are summarised in Supplementary Table 1.
Starting from our previous model26 , further supervised training improved detection of annotations and meta-annotations such as experiencer (is the concept annotated experienced by the patient or other), negation (is the concept annotated negated or not) and temporality (is the concept annotated in the past or present) with MedCATTrainer. Meta-annotations for hypothetical, historical and experiencer were merged into “Irrelevant” allowing us to exclude any mentions of a concept that do not directly relate to the patient currently. Performance of the MedCAT NLP pipeline for disorders mentioned in the text was evaluated on 4343 annotations in 146 clinical documents by a clinician (JT). F1 scores, precision, and recall are presented in Supplementary Table 2.
Measures
Outcome. The primary outcome was patient status at 14 days after symptom onset, or admission to hospital where symptom onset was missing, categorised as transfer to ICU/death (WHO-COVID-19 Outcomes Scales 6-8) vs. not ICU/death (Scales 3-5). The WHO-COVID-19 Outcome Scales 6-7 incorporate admission to an ICU while Outcome Scale 8 indicates death. Date of symptom onset, date of ICU transfer and date of death were ascertained and verified manually by a clinician.
Blood parameters . We focused on biomarkers that were routinely obtained at or shortly after admission and were therefore available for the vast majority of patients. These comprised: albumin (g/L), alanine aminotransferase (ALT; IU/L), creatinine (μmol/L), C-reactive protein (CRP; mg/L), estimated Glomerular Filtration Rate (eGFR; mL/min), Haemoglobin (g/L), lymphocyte count (x 109/L), neutrophil count (x 109/L), and platelet count (PLT; x 109/L). We also derived the neutrophil-to-lymphocyteratio (NLR) and the lymphocyte-to-CRP ratio13. Troponin-T (ng/L) and Ferritin (ug/L) were included, although these measures were only available for a subset of participants. D-dimers and HbA1c were excluded since they were measured in very few patients at admission and insufficient samples were available for analysis.
Physiological parameters. We included the six physiological parameters that form the basis of the NEWS2 score, namely, respiratory rate (breaths per minute), oxygen saturation (%), systolic blood pressure (mmHg), heart rate (beats/min), temperature (°C), and consciousness (measured by Glasgow Coma Scale (GCS) total score). All were measured at or shortly after admission. We assessed these parameters individually as well as a NEWS2 total score. Diastolic blood pressure, which is not part of the NEWS2 score, was also included in the analyses.
Demographics and comorbidities. Age, sex, ethnicity and comorbidities were considered. Where ethnicity data was available this was categorised as caucasian vs. BAME (Black, Asian and minority ethnic). For supplementary models adjusting for ethnicity, patients with ethnicity reported as ‘unknown/mixed/other’ were excluded. We included binary measures (present vs. not present) of relevant comorbid chronic health conditions derived from the NLP pipeline described above: hypertension, diabetes, heart disease (heart failure and ischemic heart disease), respiratory disease (asthma and chronic obstructive pulmonary disease, COPD) and chronic kidney disease .
Statistical analyses
Preliminary descriptive and exploratory analyses were performed. To address our first objective – exploring independent associations of physiological and blood parameters with 14-day death/ICU – we used penalised maximum likelihood logistic regression which reduces bias due to small sample size27. Each parameter was tested independently, adjusted for age and sex (Model 1) and then additionally adjusted for comorbidities (Model 2). Parameters exhibiting skewed distributions were transformed before modelling with logarithmic or square-root transformations. All parameters were scaled (mean = 0, standard deviation = 1) to improve interpretability. Outlying high values for some blood parameters were retained after individual examination by clinicians who ascertained their plausibility. We used the maximal available sample when testing each parameter. Given the number of tests conducted, P-values were adjusted using the Benjamini-Hochberg procedure to keep the False discovery rate at 5%28. These models were conducted with R 3.623 using the logistf24 package.
To address our second and third objectives – which combination of parameters performed best in predicting the 14-day outcome over and above NEWS2 – we estimated models combining all parameters using regularized logistic regression with a LASSO (Least Absolute Shrinkage and Selection Operator) estimator which shrinks parameters according to their variance, reduces over fitting and enables automatic variable selection29. The optimal degree of regularization was determined by identifying a tuning parameter λ using cross-validation30. LASSO regression provides a sparse, interpretable model, which allows us to predict individual risk scores (i.e. probability of severe outcome). Starting from an initial model with NEWS2 total score only, sets of features were added in order of (i) age and sex, (ii) blood and physiological parameters; (iii) comorbid conditions. A final model was estimated using NEWS2 total score alongside the top five most influential features from previous models. To estimate the predictive performance of our model on new unseen cases of the same underlying population, we performed internal nested cross-validation (10 folds and 20 repeats for the inner loop; 10 folds and 100 repeats for the outer loop). Overall discrimination was assessed based on the area under the curve (AUC). All continuous features were scaled (mean = 0, standard deviation = 1). Missing feature information was imputed (after scaling) using k-Nearest Neighbours imputation (k=5). Scaling and kNN imputation were incorporated within the model development and selection process to avoid data leakage which would otherwise result in optimistic performance measures31.
To assess whether a more complex machine learning estimator would improve predictive performance, we repeated this set of models using gradient boosted trees implemented in the XGBoost library32. Procedures for internally validating these models were equivalent to those described above for regularized logistic regression except the imputation step was omitted due to the ability of XGBoost to handle missing data.
The predictive performance of the derived regularized logistic regression model was then evaluated by temporal external validation33 with a hold-out sample of 256 patients who were admitted to hospital after the training sample (see Supplementary Figure 1). This involved estimating the original model exactly as presented, including scaling and imputation models derived in the training data set. Discrimination performance was assessed using AUC, sensitivity, specificity, positive predictive value (PPV) and negative predictive value (NPV). Model calibration was assessed using a calibration plot (model predicted probability vs. true probability). These models were estimated in Python 3.634 using NumPy35, and Scikit-Learn36.
Sensitivity analyses were performed to account for potential demographic variability. Recent evidence suggest sex differences with men more likely to experience worse outcomes16. Therefore, in separate models, we tested interactions between each physiological and blood parameter and sex using likelihood-ratio tests (comparing a null model with the main effects only vs. a model additionally including the interaction term). In addition, we replicated all models with adjustment for ethnicity in the subset of individuals with available data for ethnicity (n=285 in training sample).
Results
The initial inpatient cohort comprised 452 inpatients testing positive for COVID-19 of whom 159 (35%) were transferred to ICU or died (COVID-19 WHO Score 6-8) within 14 days of symptom onset. Table 1 describes the clinical characteristics of the cohort: the mean age was 67 years (standard deviation = 18.5); 54% (n=248) were male; 42% (n=120) were categorised as BAME. Patients associated with a more severe outcome were significantly older (71 vs. 65 years; p = 0.004) but there was no evidence of differences by sex or ethnicity. There were some differences between groups in the prevalence of comorbidities but these did not reach statistical significance after multiple testing correction. For example, compared to patients with less severe outcomes, those who transferred to ICU or died had higher rates of hypertension (60% vs. 50%; p = 0.11), diabetes (38% vs. 32%; p = 0.33), heart failure (16% vs. 11%; p = 0.33) and chronic kidney disease (24% vs. 16%; p = 0.11). Rates of other comorbidities were similar between the two groups. There were differences between outcome groups for most blood and physiological parameters. Patients who had transferred to ICU or died within 14 days had, at admission, lower levels of Albumin, ALT, and estimated GFR; and elevated levels of CRP, creatinine, Ferritin, and Neutrophils. Mean NEWS2 total scores were significantly different (3.4 vs 2.1; p < 0.001; corresponding to Cohen’s d of -0.57) in patients who transferred to ICU or died, compared to inpatients experiencing less severe outcomes.
Logistic regression models were used to assess independent associations between each physiological and blood parameter and disease severity measured as transfer to ICU or death (Table 2). Individuals were more likely to have transferred to ICU/died within 14 days of symptom onset if: they had higher CRP, NEWS2 score, heart rate, neutrophils, neutrophil-lymphocyte ratio, respiration rate; or if they had lower lymphocyte/CRP ratios, eGFR, creatinine, and oxygen saturation. These associations remained after adjustment for age, sex and comorbidities. There was no evidence of differences by sex (results not presented) and findings were consistent when additionally adjusting for ethnicity in secondary analyses using the subset of individuals with ethnicity data (Supplementary Table 3).
Combining physiological and blood parameters to assess ability to improve on NEWS2 in predicting 14-day outcome
To identify which minimal set of parameters were best able to improve on NEWS2 in predicting the 14-day outcome (ICU/death vs. not ICU/death), we combined all predictors in a single logistic regression model using LASSO regularisation. Internally validated predictive performance based on the area under the ROC curve (AUC) is presented in Table 3 for different feature sets. NEWS2 shows poor discrimination with an AUC of 0.628. Adding age and sex to a baseline model of NEWS2 total score only increased the AUC by 0.025 to 0.653 (+/- 2SD range: 0.639, 0.667). Further adding in all other blood and physiological parameters (except NEWS2) increased the AUC further by 0.089, to 0.742 (+/- 2SD: 0.726, 0.758). Additionally including comorbidities in this model did not improve performance. A final model was estimated including NEWS2 and the top five most important features taken from Model 4. This simpler model resulted in a slightly larger AUC of 0.751 (+/- 2SD range: 0.737, 0.764) which may indicate some overfitting due to the pre-selection of variables from previous analyses. Results were consistent when repeating these models in the subset of patients with information available on ethnicity (Supplementary Table 5).
Figure 1 summarises feature importances from the LASSO logistic regression models. When adding blood and physiological parameters to NEWS2 (‘NEWS2 + DBP’), 8 features were retained, in order of effect sizes: NEWS2 total score, CRP, neutrophils, estimated GFR, albumin, age, Troponin T, and oxygen saturation. Notably, when additionally considering comorbid conditions (‘NEWS2 + DBPC’), the retained features were similar, and no comorbid conditions were retained. This suggests that most of the variance is already captured by the top 5 parameters.
When these models were repeated using a more complex estimator (gradient boosted trees, using XGBoost32) the pattern of results was consistent with those from regularized logistic regression (Supplementary Table 5). Namely, the internally validated AUC improved from 0.646 for a model with NEWS2 alone, to 0.722 for a model that additionally included the five parameters: CRP, neutrophils, estimated GFR, albumin, and age. Importantly, while the pattern of results was consistent, a more complex machine learning estimator produced no improvements to predictive performance.
Temporal external validation was conducted on a hold-out sample of 256 patients. This sample was similar to the training sample on all parameters (Supplementary Table 6) except the proportion who transferred to ICU or died was lower. Overall, results from the hold-out sample were consistent with those from internal validation. The AUC for NEWS2 alone was 0.700, and this improved to 0.730 when adding all blood and physiological parameters (sensitivity = 0.441; specificity = 0.873). The AUC for the simplified final model including NEWS2 and the top five features (CRP, neutrophils, estimated GFR, albumin and age) was similar (AUC = 0.730; sensitivity = 0.458; specificity = 0.873) (Supplementary Table 7). Calibration for these models (Supplementary Figure 2) was acceptable but showed some consistent overestimation of risk probabilities.
Discussion
To our knowledge our study is the first to systematically attempt to improve performance of NEWS2 specifically for COVID-19. We found that the NEWS2 score shows overall poor discrimination with high specificity but poor sensitivity for severe outcomes in COVID-19 infection (transfer to ICU or death). However, its value for risk stratification (especially sensitivity) can be significantly improved by adding age and a small number of additional blood parameters (CRP, neutrophils, estimated GFR and albumin). A number of blood measures previously linked with more severe outcomes – such as lymphocyte and ALT14, or transformations of inflammatory markers such as CRP/lymphocyte or neutrophil/lymphocyte ratio – did not provide additional value to the model over and above the existing features despite being more common in those individuals with more severe outcomes. Moreover, cardiac disease and myocardial injury has been described to be commonly seen in the severe COVID-19 cases in China1,21. In our model, blood Troponin-T, a marker of myocardial injury, had additional salient signal but was only measured in a subset of our cohort at admission, so it was not included in our final model. This would have to be explored further in larger datasets. A systematic review of 10 prediction models for mortality in COVID-19 infection10 found broad similarities with the features retained in our models, particularly regarding CRP and neutrophil levels. However, existing prediction models suffer several methodological weaknesses including over-fitting, selection bias, and reliance on cross-sectional data without accounting for censoring. Additionally, almost all existing studies have relied on ethnically homogenous Chinese cohorts and thus may be unrepresentative of other global populations.
With regards to pre-existing disease comorbidities (hypertension, diabetes mellitus, heart failure, ischaemic heart disease, COPD, asthma and chronic kidney disease), these were more common in patients with severe outcomes but had minimal contribution to the risk prediction and were not retained in the final model. This was unexpected and suggests potential shared variance between pre-existing health conditions and some of the included blood or physiological markers. Future research should explore further the potential underlying shared mechanisms that can predict deterioration.
NEWS2 is a summary score derived from six physiological parameters, including oxygen saturation. While NEWS2 total score was one of the most influential parameters in our models, the oxygen saturation sub-parameter remained influential and was retained following regularisation (i.e. model ‘NEWS + DBP’). This suggests some residual association over and above what is captured by the NEWS2 score between oxygen saturation and more severe outcomes, and reinforces Royal College of Physicians guidance that the NEWS2 score ceilings with respect to respiratory function37.
Strengths and limitations
Our study included data from a large sample of patients admitted to hospital with high rates of the primary outcome (transfer to ICU or death) and considered a large number of potential predictors including demographics, physiological and blood parameters and comorbidities. However, some limitations should be acknowledged. First, there are likely to be other parameters not measured in this study that could improve the risk stratification model substantially (e.g. radiological features, other comorbidities or comorbidity load). This could be addressed by future work to introduce additional data modalities, but these were not considered in the present study to avoid limiting the real-world implementation of the risk stratification model; a complex model with many parameters will be harder to implement in clinical practice. Second, we used a 14-day time window from the symptom onset date as this provides a balance between medium-term prognostication and actionable risk stratification at the usual period of deterioration. Longer timeframes may be useful for prognostication but are harder to generalise due to the greater number of factors affecting outcomes, including institutional, regional or national policies. Since NEWS2 score is optimised for very near-term deterioration at 24 hours7, a 14-day window was used as acompromise. Third, while the hold-out sample used for temporal external validation was similar in terms of demographics, blood and physiological parameters, the rate of more severe outcomes differed significantly. Perhaps due to changes in hospital procedures over time, this again suggests the need to validate these models in other hospitals or regions. Finally, while the model was derived from two hospital sites providing a mixed population, this study highlights that initial prediction models still have poor sensitivity and recalibration would be required before implementation as a risk model in clinical practice. Validation across datasets from a wider geographical region will be necessary to ensure generalisability.
Conclusion
In conclusion, this study suggests that the simple addition of a limited number of blood parameters to the existing and widely implemented NEWS2 system can contribute to improved risk stratification among COVID-19 patients. Our model can be easily implemented in clinical practice and predicted risk score probabilities of individual patients are easy to communicate. The additional parameters are widely collected on patients at hospital admission, and with near universal usage of NEWS2 in NHS Trusts since March 201913, a minor adaptation to NEWS2 is substantially easier to implement in a variety of health settings than a bespoke risk score.
Acknowledgements
DMB is funded by a UKRI Innovation Fellowship as part of Health Data Research UK MR/S00310X/1 (https://www.hdruk.ac.uk).
RB is funded in part by grant MR/R016372/1 for the King’s College London MRC Skills Development Fellowship programme funded by the UK Medical Research Council (MRC, https://mrc.ukri.org) and by grant IS-BRC-1215-20018 for the National Institute for Health Research (NIHR, https://www.nihr.ac.uk) Biomedical Research Centre at South London and Maudsley NHS Foundation Trust and King’s College London.
AF is supported by grant IS-BRC-1215-20018 for the National Institute for Health Research (NIHR, https://www.nihr.ac.uk) Biomedical Research Centre at South London and Maudsley NHS Foundation Trust and King’s College London and The National Institute for Health Research University College London Hospitals Biomedical Research Centre.
RJBD is supported by: 1. Health Data Research UK, which is funded by the UK Medical Research Council, Engineering and Physical Sciences Research Council, Economic and Social Research Council, Department of Health and Social Care (England), Chief Scientist Office of the Scottish Government Health and Social Care Directorates, Health and Social Care Research and Development Division (Welsh Government), Public Health Agency (Northern Ireland), British Heart Foundation and Wellcome Trust. 2. The BigData@Heart Consortium, funded by the Innovative Medicines Initiative-2 Joint Undertaking under grant agreement No. 116074. This Joint Undertaking receives support from the European Union’s Horizon 2020 research and innovation programme and EFPIA; it is chaired by DE Grobbee and SD Anker, partnering with 20 academic and industry partners and ESC. 3. The National Institute for Health Research University College London Hospitals Biomedical Research Centre. 4. National Institute for Health Research (NIHR) Biomedical Research Centre at South London and Maudsley NHS Foundation Trust and King’s College London.
KO’G is supported by an MRC Clinical Training Fellowship.
RZ is supported by a King’s Prize Fellowship.
AS is supported by a King’s Medical Research Trust studentship.
KO is supported by grant MR/R017751/1
AMS is supported by the British Heart Foundation (CH/1999001/11735), the National Institute for Health Research (NIHR) Biomedical Research Centre at Guy’s & St Thomas’ NHS Foundation Trust and King’s College London (IS-BRC-1215-20006), and the Fondation Leducq.
AP is partially supported by NIHR NF-SI-0617-10120.
This work was supported by the National Institute for Health Research (NIHR) University College London Hospitals (UCLH) Biomedical Research Centre (BRC) Clinical and Research Informatics Unit (CRIU), NIHR Health Informatics Collaborative (HIC), and by awards establishing the Institute of Health Informatics at University College London (UCL). This work was also supported by Health Data Research UK, which is funded by the UK Medical Research Council, Engineering and Physical Sciences Research Council, Economic and Social Research Council, Department of Health and Social Care (England), Chief Scientist Office of the Scottish Government Health and Social Care Directorates, Health and Social Care Research and Development Division (Welsh Government), Public Health Agency (Northern Ireland), British Heart Foundation and the Wellcome Trust.
This paper represents independent research part funded by the National Institute for Health Research (NIHR) Biomedical Research Centre at South London and Maudsley NHS Foundation Trust and King’s College London. The views expressed are those of the author(s) and not necessarily those of the NHS, the NIHR or the Department of Health and Social Care. The funders had no role in study design, data collection and analysis, decision to publish, or preparation of the manuscript. We would also like to thank all the clinicians managing the patients, the patient experts of the KERRI committee, Professor Irene Higginson, Professor Alastair Baker, Professor Jules Wendon, Dan Persson and Damian Lewsley for their support.
References
- Zhou F, Yu T, Du R, et al. Clinical course and risk factors for mortality of adult inpatients with COVID-19 in Wuhan, China: a retrospective cohort study. The Lancet. 2020;395(10229):1054-1062. doi:10.1016/S0140-6736(20)30566-3
- WHO. WHO COVID-19 Dashboard. https://who.sprinklr.com/. Published 2020. Accessed April 20, 2020.
- Scott LJ, Redmond NM, Tavaré A, Little H, Srivastava S, Pullyblank A. Association between National Early Warning Scores in primary care and clinical outcomes: an observational study in UK primary and secondary care. Br J Gen Pract. April 2020. doi:10.3399/bjgp20X709337
- Lambden S, Laterre PF, Levy MM, Francois B. The SOFA score—development, utility and challenges of accurate assessment in clinical trials. Crit Care. 2019;23(1):374. doi:10.1186/s13054-019-2663-7
- Lim WS, Eerden MM van der, Laing R, et al. Defining community acquired pneumonia severity on presentation to hospital: an international derivation and validation study. Thorax. 2003;58(5):377-382. doi:10.1136/thorax.58.5.377
- Royal College of Physicians. National Early Warning Score (NEWS) 2: Standardising the Assessment of Acute-Illness Severity in the NHS. Updated Report of a Working Party. London: RCP; 2017.
- Smith GB, Prytherch DR, Meredith P, Schmidt PE, Featherstone PI. The ability of the National Early Warning Score (NEWS) to discriminate patients at risk of early cardiac arrest, un anticipated intensive care unit admission, and death. Resuscitation. 2013;84(4):465-470. doi:10.1016/j.resuscitation.2012.12.016
- Ji D, Zhang D, Xu J, et al. Prediction for Progression Risk in Patients with COVID-19 Pneumonia: the CALL Score. Clin Infect Dis. doi:10.1093/cid/ciaa414
- Shi Y, Yu X, Zhao H, Wang H, Zhao R, Sheng J. Host susceptibility to severe COVID-19 and establishment of a host risk score: findings of 487 cases outside Wuhan. Crit Care. 2020;24(1):108. doi:10.1186/s13054-020-2833-7
- Wynants L, Calster BV, Bonten MMJ, et al. Prediction models for diagnosis and prognosis of covid-19 infection: systematic review and critical appraisal. BMJ. 2020;369. doi:10.1136/bmj.m1328
- COVIDAnalytics. https://www.covidanalytics.io/calculator. Accessed April 21, 2020.
- Liao X, Wang B, Kang Y. Novel coronavirus infection during the 2019–2020 epidemic: preparing intensive care units—the experience in Sichuan Province, China. Intensive Care Med. 2020;46(2):357-360. doi:10.1007/s00134-020-05954-2
- NHS England » National Early Warning Score (NEWS). https://www.england.nhs.uk/ourwork/clinical-policy/sepsis/nationalearlywarningscore/. Accessed April 23, 2020.
- Huang C, Wang Y, Li X, et al. Clinical features of patients infected with 2019 novel coronavirus in Wuhan, China. The Lancet. 2020;395(10223):497-506. doi:10.1016/S0140-6736(20)30183-5
- Li K, Wu J, Wu F, et al. The Clinical and Chest CT Features Associated with Severe and Critical COVID-19 Pneumonia. Invest Radiol. 2020;Publish Ahead of Print. doi:10.1097/RLI.0000000000000672
- Xie J, Tong Z, Guan X, Du B, Qiu H. Clinical Characteristics of Patients Who Died of Coronavirus Disease 2019 in China. JAMA Netw Open. 2020;3(4):e205619-e205619. doi:10.1001/jamanetworkopen.2020.5619
- Zhang J-J, Dong X, Cao Y-Y, et al. Clinical characteristics of 140 patients infected withSARS-CoV-2 in Wuhan, China. Allergy. February 2020. doi:10.1111/all.14238
- Ruan Q, Yang K, Wang W, Jiang L, Song J. Clinical predictors of mortality due to COVID-19 based on an analysis of data of 150 patients from Wuhan, China. Intensive Care Med. March 2020. doi:10.1007/s00134-020-05991-x
- Guan W, Ni Z, Hu Y, et al. Clinical Characteristics of Coronavirus Disease 2019 in China. N Engl J Med. February 2020. doi:10.1056/NEJMoa2002032
- Lagunas-Rangel FA. Neutrophil-to-lymphocyte ratio and lymphocyte-to-C-reactive proteinratio in patients with severe coronavirus disease 2019 (COVID-19): A meta-analysis. J MedVirol. April 2020. doi:10.1002/jmv.25819
- Guo T, Fan Y, Chen M, et al. Cardiovascular Implications of Fatal Outcomes of PatientsWith Coronavirus Disease 2019 (COVID-19). JAMA Cardiol. March 2020.doi:10.1001/jamacardio.2020.1017
- Jackson R, Kartoglu I, Stringer C, et al. CogStack – experiences of deploying integratedinformation retrieval and extraction services in a large National Health Service Foundation Trust hospital. BMC Med Inform Decis Mak. 2018;18. doi:10.1186/s12911-018-0623-9
- Kraljevic Z, Bean D, Mascio A, et al. MedCAT — Medical Concept Annotation Tool. ArXiv191210166 Cs Stat. December 2019. http://arxiv.org/abs/1912.10166. Accessed April 17, 2020.
- Searle T, Kraljevic Z, Bendayan R, Bean D, Dobson R. MedCATTrainer: A Biomedical FreeText Annotation Interface with Active Learning and Research Use Case Specific Customisation. ArXiv190707322 Cs. July 2019. http://arxiv.org/abs/1907.07322. Accessed April 17, 2020.
- Johnson AEW, Pollard TJ, Shen L, et al. MIMIC-III, a freely accessible critical care database. SciData. 2016;3(1):1-9.doi:10.1038/sdata.2016.35
- Bean D, Kraljevic Z, Searle T, et al. Treatment with ACE-inhibitors is associated with less severe disease with SARS-Covid-19 infection in a multi-site UK acute Hospital Trust. medRxiv. April 2020:2020.04.07.20056788. doi:10.1101/2020.04.07.20056788
- Firth D. Bias Reduction of Maximum Likelihood Estimates. Biometrika. 1993;80(1):27-38. doi:10.2307/2336755
- Benjamini Y, Hochberg Y. Controlling the False Discovery Rate – a Practical and Powerful Approach. JR Stat Soc Ser B-Methodol. 1995;57(1):289-300.
- Tibshirani R. Regression Shrinkage and Selection Via the Lasso. J R Stat Soc Ser B Methodol. 1996;58(1):267-288. doi:10.1111/j.2517-6161.1996.tb02080.x
- Hastie T, Tibshirani R, Wainwright M. Statistical Learning with Sparsity: The Lasso and Generalizations. New York: CRC Press; 2015.
- Kuhn M, Johnson K. Applied Predictive Modeling. Vol 26. Springer; 2013.
- Chen T, Guestrin C. XGBoost: A scalable tree boosting system. In: Proceedings of the 22nd ACM SIGKDD International Conference on Knowledge Discovery and Data Mining. KDD’16. New York, NY, USA: ACM; 2016:785-794. doi:10.1145/2939672.2939785
- Steyerberg E. Clinical Prediction Models. Second Edition. Cham, Switzerland: Springer; 2019.
- Van Rossum G, Drake FL. Python 3 Reference Manual. Scotts Valley, CA: Create Space; 2009.
- Oliphant T. NumPy: A guide to NumPy. 2006. http://www.numpy.org/.
- Pedregosa F, Varoquaux G, Gramfort A, et al. Scikit-learn: Machine learning in Python. J Mach Learn Res. 2011;12:2825-2830.
- NEWS2 and deterioration in COVID-19. RCP London. https://www.rcplondon.ac.uk/news/news2-and-deterioration-covid-19. Published April 14, 2020. Accessed April 24, 2020.
Tables
Table 1: Patient characteristics at hospital admission
N avail. | All patients | Patients by status at 14-day endpoint | FDR-adjusted P-value for test between outcome groups1 |
||
---|---|---|---|---|---|
WHO-COVID-19 Outcomes Scales 3-5 (no ICU/death) n=393 | WHO-COVID-19 Outcomes Scales 6-8 (ICU/death) n=159 | ||||
Age | 452 | 67.00 [28.00] | 64.87 [30.00] | 70.92 [27.00] | 0.004 |
Sex (male) N (%) | 452 | 248 (54.9%) | 157 (53.6%) | 91 (57.2%) | 0.682 |
BAME N (%) | 285 | 120 (42.1%) | 73 (41.0%) | 47 (43.9%) | 0.770 |
Comorbidities | N avail. | N (%) | P-value | ||
Hypertension | 452 | 243 (53.8%) | 147 (50.2%) | 96 (60.4%) | 0.106 |
Diabetes mellitus | 452 | 154 (34.1%) | 93 (31.7%) | 61 (38.4%) | 0.325 |
Heart Failure | 452 | 57 (12.6%) | 32 (10.9%) | 25 (15.7%) | 0.325 |
Ischaemic Heart Diseases | 452 | 85 (18.8%) | 55 (18.8%) | 30 (18.9%) | 1.000 |
COPD | 452 | 48 (10.6%) | 27 (9.2%) | 21 (13.2%) | 0.366 |
Asthma | 452 | 65 (14.4%) | 44 (15.0%) | 21 (13.2%) | 0.770 |
Chronic Kidney Disease | 452 | 84 (18.6%) | 46 (15.7%) | 38 (23.9%) | 0.105 |
Blood biomarkers | N avail. | Mean [IQR] | P-value | ||
Albumin | 322 | 37.11 [7.00] | 38.05 [7.00] | 35.48 [7.00] | <0.001 |
Alanine aminotransferase (ALT) | 184 | 54.83 [33.00] | 60.34 [30.50] | 46.45 [34.00] | 0.386 |
C-reactive protein (CRP) | 419 | 93.55 [106.70] | 72.99 [84.90] | 130.41 [135.62] | <0.001 |
Creatinine | 420 | 121.67 [49.00] | 105.86 [40.50] | 150.42 [72.00] | 0.001 |
Estimated GFR | 334 | 63.75 [40.00] | 68.01 [36.00] | 56.05 [44.50] | <0.001 |
Ferritin | 122 | 1356.01 [1165.25] | 1272.35 [1149.75] | 1442.45 [902.50] | 0.016 |
Haemoglobin | 419 | 125.05 [30.00] | 125.52 [30.00] | 124.21 [28.75] | 0.770 |
Lymphocyte count | 419 | 1.45 [0.67] | 1.10 [0.69] | 2.09 [0.67] | 0.695 |
Neutrophil count | 418 | 5.72 [3.53] | 5.06 [3.01] | 6.91 [5.31] | <0.001 |
Neutrophil/lymphocyte ratio | 418 | 6.80 [5.01] | 5.81 [4.22] | 8.58 [6.26] | <0.001 |
Lymphocyte/CRP ratio | 416 | 0.07 [0.04] | 0.08 [0.05] | 0.05 [0.02] | <0.001 |
Platelet count | 421 | 226.68 [103.00] | 228.34 [102.50] | 223.69 [104.25] | 0.958 |
Troponin T | 141 | 33.92 [29.00] | 30.40 [26.00] | 37.92 [38.50] | 0.351 |
Physiological parameters | N avail. | Mean [IQR] | P-value | ||
NEWS2 Total Score | 401 | 2.51 [3.00] | 2.10 [3.00] | 3.40 [4.00] | <0.001 |
Heart rate | 405 | 85.35 [20.00] | 84.49 [19.00] | 87.15 [23.50] | 0.359 |
Oxygen saturation | 404 | 96.22 [3.00] | 96.54 [2.00] | 95.56 [3.00] | 0.008 |
Respiration rate | 405 | 19.84 [2.00] | 19.42 [2.00] | 20.72 [3.00] | 0.008 |
GCS score | 172 | 14.12 [1.00] | 14.20 [1.00] | 13.95 [1.00] | 0.117 |
Systolic blood pressure | 405 | 127.39 [29.00] | 127.09 [26.50] | 128.00 [32.00] | 0.770 |
Diastolic blood pressure | 405 | 72.69 [18.00] | 73.20 [18.00] | 71.63 [19.00] | 0.325 |
Temperature | 405 | 37.12 [0.90] | 37.12 [0.90] | 37.11 [1.00] | 0.682 |
Notes.
[1] Wilcoxon test for continuous variables; Χ2 test for binary variables. FDR-corrected P-values based on the Benjamini–Hochberg correction.
Table 2: Logistic regression models for each blood and physiological measure tested separately, sorted by effect size
N avail. | Model 1: Age, sex only | Model 2: + all comorbidities | |||
---|---|---|---|---|---|
Odds Ratio [95% C.I.] | FDR-adjusted P-value[1] | Odds Ratio [95% C.I.] | FDR-adjusted P-value[1] |
||
CRP | 419 | 2.04 [1.64, 2.57] | <0.001 | 2.06 [1.65, 2.60] | <0.001 |
NEWS2 Total Score | 401 | 1.82 [1.46, 2.30] | <0.001 | 1.83 [1.46, 2.31] | <0.001 |
Lymphocyte/CRP ratio | 416 | 0.56 [0.44, 0.71] | <0.001 | 0.56 [0.44, 0.71] | <0.001 |
Troponin T | 141 | 1.51 [1.02, 2.30] | 0.119 | 1.69 [1.08, 2.78] | 0.073 |
Neutrophil count | 418 | 1.66 [1.33, 2.09] | <0.001 | 1.68 [1.35, 2.12] | <0.001 |
Ferritin | 122 | 1.55 [1.05, 2.40] | 0.098 | 1.60 [1.07, 2.54] | 0.073 |
Estimated GFR | 334 | 0.65 [0.51, 0.83] | 0.004 | 0.66 [0.49, 0.87] | 0.023 |
Respiration rate | 405 | 1.47 [1.19, 1.83] | 0.002 | 1.46 [1.19, 1.82] | 0.003 |
Albumin | 322 | 0.68 [0.53, 0.87] | 0.010 | 0.69 [0.53, 0.89] | 0.024 |
Oxygen saturation | 404 | 0.72 [0.57, 0.89] | 0.010 | 0.71 [0.56, 0.88] | 0.013 |
Neutrophil/lymphocyte ratio | 418 | 1.35 [1.09, 1.70] | 0.026 | 1.36 [1.09, 1.72] | 0.028 |
Creatinine | 420 | 1.35 [1.09, 1.69] | 0.024 | 1.35 [1.04, 1.76] | 0.073 |
Heart rate | 405 | 1.30 [1.05, 1.62] | 0.068 | 1.32 [1.06, 1.65] | 0.050 |
ALT | 184 | 1.17 [0.86, 1.60] | 0.923 | 1.22 [0.88, 1.68] | 0.682 |
Temperature | 405 | 1.09 [0.88, 1.36] | 1.000 | 1.10 [0.88, 1.36] | 0.999 |
Diastolic blood pressure | 405 | 0.90 [0.73, 1.11] | 0.952 | 0.92 [0.74, 1.13] | 0.999 |
Platelet count | 421 | 0.95 [0.77, 1.16] | 1.000 | 0.94 [0.76, 1.15] | 0.999 |
Lymphocyte count | 419 | 1.05 [0.86, 1.29] | 1.000 | 1.05 [0.86, 1.29] | 0.999 |
GCS score | 172 | 0.95 [0.70, 1.31] | 1.000 | 0.96 [0.70, 1.32] | 0.999 |
Hemoglobin | 419 | 0.98 [0.79, 1.20] | 1.000 | 1.03 [0.83, 1.27] | 0.999 |
Systolic blood pressure | 405 | 0.97 [0.78, 1.20] | 1.000 | 0.98 [0.78, 1.21] | 0.999 |
Notes.
[1] FDR-corrected P-values based on the Benjamini–Hochberg correction. Odds ratios represent a one standard deviation change in the respective blood and clinical measure at admission (tested in separate models). Model 1 adjusted for age and sex. Model 2 additionally adjusted for comorbidities (hypertension, diabetes, heart diseases, respiratory diseases and chronic kidney disease).
Table 3: Internally validated predictive performance (n=452)
Notes. AUC based on repeated, nested cross-validation (inner loop: 10-fold, 20 repeats; outer loop = 10-fold, 100 repeats). Missing values imputed at each outer loop with k-Nearest Neighbours (KNN) imputation.
Included features | Internally validated AUC | Sensitivity | Specificity | PPV | NPV | |||
---|---|---|---|---|---|---|---|---|
Mean | -2SD | +2SD | ||||||
1 | NEWS2 | 0.628 | 0.619 | 0.637 | 0.18 | 0.95 | 0.664 | 0.681 |
2 | NEWS2 + D | 0.653 | 0.639 | 0.667 | 0.189 | 0.929 | 0.597 | 0.678 |
3 | NEWS2 + DBP | 0.742 | 0.726 | 0.758 | 0.4 | 0.857 | 0.585 | 0.723 |
4 | NEWS2 + DBPC | 0.737 | 0.721 | 0.753 | 0.385 | 0.854 | 0.588 | 0.719 |
5 | NEWS2 + CRP + Neutrophil + eGFR + Albumin + Age | 0.751 | 0.737 | 0.764 | 0.415 | 0.842 | 0.589 | 0.727 |
D = Age, sex
C = comorbidities (8 features) B = bloods (10 features)
P = physiological parameters (7 features)
Figures
Figure 1: Feature importances from LASSO logistic regression in training sample (n=452)
Notes. Feature importances refer to absolute values of standardised coefficients from logistic regression, sorted by effect size in model ‘NEWS2 + DBPC’. Where a feature is labelled on the y-axis, it was entered into the model. Features retained following LASSO regularisation are represented by a coloured bar; the absence of a bar indicates that this feature was omitted during regularisation.
Supplementary Materials
Supplementary Table 1: SNOMED terms
SNOMED concept name | SNOMED concept IDs |
---|---|
Diabetes | S-230572002, S-44054006, S-237599002, S-49455004 |
Heart Failure | S-42343007, S-426263006, S-48447003, S-418304008, S-10633002 |
IHD | S-401314000, S-194828000, S-233839009, S-414545008, S-394659003, S-1755008, S-413838009 |
Hypertension | S-59621000 |
COPD | S-13645005, S-313297008 |
Asthma | S-195967001 |
CKD | S-433144002, S-90688005, S-709044004 |
Supplementary Table 2: F1, precision and recall for NLP co-morbidity detection
MedCATTrainer24 was used to collect manual annotations for 146 clinical documents totalling 4343 annotations. Each co-morbidity is defined using one or more SNOMED terms. Predicted true positive labels (TP), precision (P), recall (R), F1-score (F1) are shown for these aggregated concepts. These results only consider entity detection and not meta annotation.
TP | F1 | P | R | SNOMED terms | |
---|---|---|---|---|---|
Diabetes mellitus | 73 | 0.936 | 0.924 | 0.948 | S-230572002, S-44054006, S-237599002, S-49455004 |
Heart Failure | 11 | 0.893 | 0.786 | 1.000 | S-42343007, S-426263006, S-48447003, S-418304008, S-10633002 |
IHD | 23 | 0.979 | 0.958 | 1.000 | S-401314000, S-194828000, S-233839009, S-414545008, S-394659003, S-1755008, S-413838009 |
Hypertension | 84 | 0.883 | 0.988 | 0.778 | S-59621000 |
COPD | 14 | 0.967 | 0.933 | 1.000 | S-13645005, S-313297008 |
Asthma | 11 | 1.000 | 1.000 | 1.000 | S-195967001 |
CKD | 15 | 0.938 | 0.938 | 0.938 | S-433144002, S-90688005, S-709044004 |
Supplementary Table 3: Logistic regression models for each blood measure tested separately, adjusted for ethnicity for patients with information on ethnicity
Measure | N avail. | Model 1: Age, sex, ethnicity | Model 2: + all comorbidities | ||
---|---|---|---|---|---|
OR [95% C.I.] | FDR-adjusted P-value | OR [95% C.I.] | FDR-adjusted P-value |
||
CRP | 263 | 2.15 [1.63, 2.91] | <0.001 | 2.24 [1.68, 3.07] | <0.001 |
NEWS2 Total Score | 250 | 2.06 [1.56, 2.79] | <0.001 | 2.04 [1.54, 2.77] | <0.001 |
Troponin T | 84 | 1.62 [0.94, 2.99] | 0.394 | 1.86 [1.01, 3.60] | 0.210 |
Lymphocyte/CRP ratio | 260 | 0.57 [0.41, 0.76] | 0.001 | 0.56 [0.41, 0.76] | 0.001 |
Neutrophil count | 262 | 1.57 [1.20, 2.12] | 0.007 | 1.56 [1.19, 2.10] | 0.009 |
Oxygen saturation | 252 | 0.63 [0.47, 0.83] | 0.009 | 0.66 [0.49, 0.87] | 0.022 |
Heart rate | 253 | 1.46 [1.12, 1.93] | 0.029 | 1.45 [1.11, 1.92] | 0.037 |
Respiration rate | 253 | 1.46 [1.15, 1.90] | 0.012 | 1.44 [1.14, 1.87] | 0.021 |
GCS score | 109 | 0.70 [0.43, 1.11] | 0.440 | 0.70 [0.42, 1.14] | 0.527 |
Albumin | 191 | 0.71 [0.51, 0.97] | 0.162 | 0.71 [0.51, 0.99] | 0.210 |
Creatinine | 264 | 1.24 [0.95, 1.65] | 0.440 | 1.33 [0.97, 1.87] | 0.341 |
Estimated GFR | 199 | 0.81 [0.58, 1.11] | 0.594 | 0.77 [0.53, 1.12] | 0.553 |
ALT | 130 | 1.14 [0.73, 1.80] | 1.000 | 1.26 [0.79, 2.04] | 0.950 |
Neutrophil/lymphocyte ratio | 262 | 1.24 [0.95, 1.65] | 0.440 | 1.22 [0.94, 1.62] | 0.527 |
Temperature | 253 | 1.18 [0.92, 1.52] | 0.594 | 1.18 [0.92, 1.53] | 0.573 |
Ferritin | 81 | 1.08 [0.64, 1.81] | 1.000 | 1.17 [0.69, 2.00] | 1.000 |
Platelet count | 265 | 0.89 [0.67, 1.15] | 1.000 | 0.89 [0.67, 1.15] | 1.000 |
Diastolic blood pressure | 253 | 0.89 [0.67, 1.17] | 1.000 | 0.91 [0.68, 1.20] | 1.000 |
Lymphocyte count | 263 | 1.08 [0.85, 1.37] | 1.000 | 1.08 [0.85, 1.38] | 1.000 |
Hemoglobin | 265 | 1.07 [0.83, 1.38] | 1.000 | 1.07 [0.83, 1.40] | 1.000 |
Systolic blood pressure | 253 | 0.90 [0.69, 1.17] | 1.000 | 0.93 [0.71, 1.21] | 1.000 |
Notes.
Odds ratios for 1 SD change in each blood measure at admission (tested in separate models) Model 1 adjusted for age and sex and ethnicity. Model 2 additionally adjusted for comorbidities (hypertension, diabetes, heart diseases, respiratory diseases and chronic kidney disease)
Supplementary Table 4: Internally validated predictive performance, adjusted for ethnicity for patients with information on ethnicity (n=285)
Included features | Internally validated AUC | Sensitivity | Specificity | PPV | NPV | |||
---|---|---|---|---|---|---|---|---|
Mean | -2SD | +2SD | ||||||
1 | NEWS2 | 0.663 | 0.641 | 0.648 | 0.256 | 0.889 | 0.582 | 0.665 |
2 | NEWS2 + D | 0.654 | 0.628 | 0.68 | 0.283 | 0.878 | 0.585 | 0.671 |
3 | NEWS2 + DBP | 0.722 | 0.693 | 0.75 | 0.432 | 0.805 | 0.571 | 0.702 |
4 | NEWS2 + DBPC | 0.71 | 0.681 | 0.74 | 0.434 | 0.794 | 0.559 | 0.7 |
5 | NEWS2 + CRP + Neutrophil + eGFR + Albumin + Age | 0.734 | 0.713 | 0.756 | 0.414 | 0.797 | 0.549 | 0.693 |
D = Age, sex
C = comorbidities (8 features)
B = bloods (10 features)
P = physiological parameters (7 features)
Supplementary Table 5: Internally validated predictive performance using XGBoost (Gradient Boosting Trees) (n=452)
AUC based on repeated, nested cross-validation (inner loop: 10-fold, 20 repeats; outer loop = 10-fold, 100 repeats).
Included features | Internally validated AUC | Sensitivity | Specificity | PPV | NPV | |||
---|---|---|---|---|---|---|---|---|
Mean | -2SD | +2SD | ||||||
1 | NEWS2 | 0.646 | 0.626 | 0.666 | 0.364 | 0.880 | 0.624 | 0.718 |
2 | NEWS2 + D | 0.667 | 0.652 | 0.682 | 0.344 | 0.910 | 0.680 | 0.719 |
3 | NEWS2 + DBP | 0.728 | 0.700 | 0.755 | 0.452 | 0.837 | 0.601 | 0.739 |
4 | NEWS2 + DBPC | 0.719 | 0.693 | 0.745 | 0.428 | 0.839 | 0.591 | 0.731 |
5 | NEWS2 + CRP + Neutrophil + eGFR+ + Albumin + Age | 0.722 | 0.660 | 0.785 | 0.480 | 0.836 | 0.615 | 0.748 |
D = Age, sex
C = comorbidities (8 features)
B = bloods (10 features)
P = physiological parameters (7 features)
Supplementary Table 6: Comparison of training and held-out validation samples
Training sample (n=452) | Validation sample (n=256) | P-value for test of difference between samples1 |
|||
---|---|---|---|---|---|
14-day outcome | N avail. | N (%) | N avail. | N (%) | |
COVID-19 WHO Score 6-8 (ICU/death) | 452 | 159 (35.2%) | 256 | 59 (23.0%) | 0.001 |
Demographics | |||||
Age | 452 | 67.0 [28.0] | 256 | 67.9 [25.5] | 0.822 |
Sex (male) N (%) | 452 | 248 (54.9%) | 256 | 137 (53.5%) | 0.788 |
BAME N (%) | 285 | 120 (42.1%) | 206 | 86 (41.7%) | 0.999 |
Comorbidities | N avail. | N (%) | P-value | ||
Hypertension | 452 | 243 (53.8%) | 256 | 146 (57.0%) | 0.446 |
Diabetes | 452 | 154 (34.1%) | 256 | 85 (33.2%) | 0.879 |
Heart Failure | 452 | 57 (12.6%) | 256 | 24 (9.4%) | 0.239 |
Ischaemic Heart Diseases | 452 | 85 (18.8%) | 256 | 43 (16.8%) | 0.572 |
COPD | 452 | 48 (10.6%) | 256 | 30 (11.7%) | 0.746 |
Asthma | 452 | 65 (14.4%) | 256 | 37 (14.5%) | 0.999 |
Chronic Kidney Disease | 452 | 84 (18.6%) | 256 | 39 (15.2%) | 0.304 |
Blood biomarkers | N avail. | Mean [IQR] | P-value | ||
Albumin | 322 | 37.1 [7.0] | 219 | 36.4 [6.0] | 0.079 |
ALT | 184 | 54.8 [33.0] | 105 | 42.8 [31.0] | 0.889 |
CRP | 419 | 93.5 [106.7] | 224 | 97.7 [94.2] | 0.341 |
Creatinine | 420 | 121.7 [49.0] | 226 | 147.1 [62.8] | 0.19 |
Estimated GFR | 334 | 63.7 [40.0] | 225 | 59.7 [44.0] | 0.076 |
Ferritin | 122 | 1356.0 [1165.2] | 78 | 1668.8 [1258.2] | 0.702 |
Haemoglobin | 419 | 125.1 [30.0] | 226 | 125.3 [31.0] | 0.919 |
Lymphocyte count | 419 | 1.5 [0.7] | 226 | 1.3 [0.6] | 0.247 |
Neutrophil count | 418 | 5.7 [3.5] | 226 | 5.7 [3.9] | 0.952 |
Neutrophil/lymphocyte ratio | 418 | 6.8 [5.0] | 226 | 6.8 [4.7] | 0.387 |
Lymphocyte/CRP ratio | 416 | 0.1 [0.0] | 224 | 0.0 [0.0] | 0.191 |
Platelet count | 421 | 226.7 [103.0] | 226 | 223.7 [124.2] | 0.652 |
Troponin T | 141 | 33.9 [29.0] | 94 | 87.8 [45.2] | 0.414 |
Physiological parameters | N avail. | Mean [IQR] | P-value | ||
NEWS2 Total Score | 401 | 2.5 [3.0] | 253 | 2.7 [3.0] | 0.283 |
Heart rate | 405 | 85.4 [20.0] | 254 | 85.3 [19.0] | 0.894 |
Oxygen saturation | 404 | 96.2 [3.0] | 254 | 96.1 [3.0] | 0.562 |
Respiration rate | 405 | 19.8 [2.0] | 254 | 20.4 [2.0] | 0.161 |
GCS score | 172 | 14.1 [1.0] | 103 | 14.3 [1.0] | 0.432 |
Systolic blood pressure | 405 | 127.4 [29.0] | 254 | 127.4 [25.0] | 0.834 |
Diastolic blood pressure | 405 | 72.7 [18.0] | 254 | 72.7 [17.0] | 0.721 |
Temperature | 405 | 37.1 [0.9] | 254 | 37.0 [0.7] | 0.101 |
Notes.
[1] Wilcoxon test for continuous variables; Χ2 test for binary variables.
Supplementary Table 7: Temporal external validation, using hold-out sample (n=256)
Included features | AUC | Sensitivity | Specificity | PPV | NPV |
---|---|---|---|---|---|
NEWS2 | 0.700 | 0.305 | 0.939 | 0.600 | 0.819 |
NEWS2 + DBP | 0.730 | 0.441 | 0.873 | 0.510 | 0.839 |
NEWS2 + CRP + Neutrophil + eGFR + Albumin + Age | 0.730 | 0.458 | 0.873 | 0.519 | 0.843 |
D = Age, sex
C = comorbidities (8 features)
B = bloods (10 features)
P = physiological parameters (7 features)
The effects of ARBs, ACEIs and statins on clinical outcomes of COVID-19 infection among nursing home residents
First online: 15-05-2020 | Last update: 18-05-2020
Authors
Anton De Spiegeleer1,2,3*, Antoon Bronselaer4*, James T Teo5, Geert Byttebier6, Guy De Tré4, Luc Belmans7, Richard Dobson8, Evelien Wynendaele1, Christophe Van De Wiele9, Filip Vandaele10, Diemer Van Dijck11, Dan Bean8, David Fedson12 and Bart De Spiegeleer1**
* Equally contributed as first authors
** Corresponding author; e-mail: bart.despiegeleer@ugent.be
Affiliations
- Drug Quality and Registration group, Faculty of Pharmaceutical Sciences, Ghent University, Belgium.
- Department of Geriatrics, Faculty of Medicine and Health Sciences, Ghent University Hospital, Belgium.
- Unit for Molecular Immunology and Inflammation, VIB-Center for Inflammation Research, Belgium.
- DDCM lab, Department of Telecommunications and Information Processing, Faculty of Engineering and Architecture, Ghent University, Belgium.
- Department of Clinical Neuroscience, Institute of Psychiatry, Psychology and Neuroscience, King’s College London, UK.
- Bioconstat BV, Ghent, Belgium.
- Department of Public Health and Primary Care, Faculty of Medicine and Health Sciences, Ghent University Hospital, Belgium.
- Department of Biostatistics and Health Informatics, Institute of Psychiatry, Psychology and Neuroscience, King’s College London, UK.
- Department of Diagnostic Sciences, Faculty of Medicine and Health Sciences, Ghent University Hospital, Belgium.
- VZW Zorg-Saam Zusters Kindsheid Jesu, Ghent, Belgium.
- Corilus Health IT Center, Ghent, Belgium.
- Sergy Haut, France.
Citation
De Spiegeleer, Anton, et al. “The effects of ARBs, ACEIs and statins on clinical outcomes of COVID-19 infection among nursing home residents.” medRxiv 2020.05.11.20096347. https://doi.org/10.1101/2020.05.11.20096347
Abstract
Background. COVID-19 infection has limited preventive or therapeutic drug options at this stage. Some of common existing drugs like angiotensin-converting enzyme inhibitors (ACEi), angiotensin II receptor blockers (ARB) and the HMG-CoA reductase inhibitors (‘statins’) have been hypothesised to impact on disease severity. However, up till now, no studies investigating this association were conducted in the most vulnerable and affected population groups, i.e. older people residing in nursing homes. The purpose of this study has been to explore the association of ACEi/ARB and/or statins with clinical manifestations in COVID-19 infected older people residing in nursing homes.
Methods and Findings. We undertook a retrospective multi-centre cohort study in two Belgian nursing homes that experienced similar COVID-19 outbreaks. COVID-19 diagnoses were based on clinical suspicion and/or viral presence using PCR of nasopharyngeal samples. A total of 154 COVID-19 positive subjects was identified. The outcomes were 1) serious COVID-19 defined as a long-stay hospital admission (length of stay ≥ 7 days) or death (at hospital or nursing home) within 14 days of disease onset, and 2) asymptomatic, i.e. no disease symptoms in the whole study-period while still being PCR diagnosed. Disease symptoms were defined as any COVID-19-related clinical symptom (e.g. coughing, dyspnoea, sore throat) or sign (low oxygen saturation and fever) for ≥ 2 days out of 3 consecutive days.
Logistic regression models with Firth corrections were applied on these 154 subjects to analyse the association between ACEi/ARB and/or statin use with the outcomes. Age, sex, functional status, diabetes and hypertension were used as covariates. Sensitivity analyses were conducted to evaluate the robustness of our statistical significant findings.
We found a statistically significant association between statin intake and the absence of symptoms during COVID-19 infection (unadjusted OR 2.91; CI 1.27-6.71; p=0.011), which remained statistically significant after adjusting for age, sex, functional status, diabetes mellitus and hypertension. The strength of this association was considerable and clinically important. Although the effects of statin intake on serious clinical outcome (long-stay hospitalisation or death) were in the same beneficial direction, these were not statistically significant (OR 0.75; CI 0.25-1.85; p=0.556). There was also no statistically significant association between ACEi/ARB and asymptomatic status (OR 1.52; CI 0.62-3.50; p=0.339) or serious clinical outcome (OR 0.79; CI 0.26-1.95; p=0.629).
Conclusions. Our data indicate that statin intake in old, frail people could be associated with a considerable beneficial effect on COVID-19 related clinical symptoms. The role of statins and any interaction with renin-angiotensin system drugs need to be further explored in larger observational studies as well as randomised clinical trials.
Introduction
Patients with serious and fatal COVID-19 infections are characterised by pneumonia-associated acute respiratory distress syndrome (ARDS) and multi-organ failure. The underlying mechanisms are linked to an imbalance between ACE and ACE2, as well as endothelial dysfunction (1-3) (Figure 1). Animal experiments have indicated that ARBs, ACEis or statins can prevent experimentally induced ARDS (4). These drugs are also likely to counteract the effects of sepsis-associated coagulopathy, elevated pro-inflammatory cytokines (e.g. IL-6) and sepsis-associated effects on pulmonary vascular permeability (5-12).
In a non-COVID-19 context, clinical investigators have observed that pneumonia patients who had been taking statins, ARBs or ACEis had improved survival (13, 14). Moreover, recent observational studies have reported similar findings for hospitalized COVID-19 patients (15-19). Recently, randomized controlled clinical trials have begun to evaluate the clinical effects of ARB, ACEi or statin treatment in hospitalized COVID-19 patients (e.g. NCT04348695, NCT04343001, NCT04351581). However, the estimated completion dates for these trials will be some time in 2021, and most will only consider ARB/ACEi monotherapy, i.e. not in combination with statins.
To our knowledge, no ARB/ACEi/statin studies have been or are being conducted among elderly nursing home residents, the most vulnerable individuals for COVID-19 morbidity and mortality. In Belgium, a country with a well-developed health care system, 3000 residents of nursing homes have died from COVID-19, with still around 100 residents a day currently dying (20). Estimates for the US suggest that almost 20% of all COVID-19 deaths have occurred in long-term care centers (21). Thus, every day without effective therapy comes at a high human cost.
We aimed to replicate the hospital findings to a frail, high-risk population living in nursing homes. While we wait for the results of prospective clinical trials, our findings allow us to make suggestions about the use of ACEis/ARBs and statins for these COVID-19 patients.
Figure 1. Three mechanisms suggested for the effects of statins and ACEis/ARBs in preventing severe pulmonary disease in COVID-19
1) Under normal conditions the Tie-2 receptor is continuously activated by Angiopoetin-1 (Angpt-1), which in turn activates Akt-kinase, leading to phosphorylation and hence inhibition of the transcription factor Foxo1. Unphosphorylated or active Foxo1 initiates the transcription of genes leading to increased inflammation, decreased endothelial barrier integrity and hypercoagulability. Angpt-2 is a partial antagonist of the Tie-2 receptor, stimulating inflammation, endothelial dysfunction and hypercoagulability. COVID-19 infection and ARDS are associated with increased Angpt-2 levels in blood, while statins simulate the Angpt-1 pathways. 2) The RAS system activates angiotensin-1 receptors (AT1R), stimulating inflammation, hypercoagulability and endothelial permeability. The Ang II-ACE2-Ang(1-7)-Mas receptor pathway counteracts the effects of this RAS system. COVID-19 enters the cell through ACE2 receptors, thereby decreasing these membrane-bound receptors, and relatively stimulating the RAS system. ACEis/ARBs inhibit the RAS system, while concomitantly increasing ACE-2 expression, which protects against ARDS. Statins also increase ACE-2 expression. 3) In ARDS there is an increase in the activation of the MyD88-NFkB inflammatory pathway. Statins preserve MyD88 at normal levels and down regulate NFkB. Black lines = stimulating effects; red lines = inhibiting effects.
Methods
This retrospective study conformed with all legal guidelines and the protocol was approved by the Ethical Committee of the Ghent University Hospital (reference BC-07671).
Study design
The retrospective study cohort was defined as all (anonymised) residents at two elderly care homes with COVID-19 diagnosis based on clinical grounds and/or PCR lab testing from 1st of March to 16th of April. Both elderly care homes experienced COVID-19 outbreaks during this period. To determine the day of disease onset, structured and unstructured diagnostic records were analysed for symptoms suggesting COVID-19 infection. The first day of suggestive symptoms on two out of three consecutive days was considered as the day of disease onset. For the PCR-diagnosed residents, the suggestive symptoms used for disease onset were cough, shortness of breath (dyspnoea), sore throat, runny nose, general weakness, headache, confusion, muscle pain, arthralgia, diarrhoea, abdominal pain, vomiting, fever (T° > 37.6°C), increased oxygen need or low oxygen saturation (SpO2 ≤ 92%). In cases where no symptoms were mentioned (while still being PCR COVID-19-positive diagnosed), the date of nasopharyngeal sampling was used as the day of disease onset. For the clinically diagnosed residents without a confirmatory PCR lab test, the symptoms used for determining disease onset were defined more strictly, i.e., respiratory complaints (cough, shortness of breath, sore throat, runny nose), fever (T° > 37.6°C), increased oxygen-need or low oxygen saturation (SpO2 ≤ 92%).
The primary outcomes were 1) serious COVID-19, i.e. long-stay hospital admission (length of stay ≥ 7 days) or death (at nursing home or hospital) within 14 days of disease onset, and 2) asymptomatic, i.e. no disease symptoms as defined above throughout the whole study-period while still being PCR diagnosed.
All residents were stratified according to drug exposure to ACEi or ARB within 7 days before the day of disease onset or during the disease (prior to an outcome being reached). Specifically, we considered as treated all residents taking ≥ 2 days an ACEi (ramipril, lisinopril, enalapril, captopril, quinapril, imidapril, fosinopril, trandolapril) or ARB (candesartan, irbesartan, losartan, olmesartan, telmisartan, valsartan) up to 7 days before or 14 days after disease onset. An identical protocol was used to stratify according to drug exposure to statins (atorvastatin, fluvastatin, pravastatin, rosuvastatin, simvastatin).
We developed a mapping table based on clinical prescriptions to determine the diabetic and hypertension status of all residents. It was designed by a specialist in elderly care and validated by two independent physicians, one a general physician and the other a cardiologist.
The functional status of all residents was a dichotomous variable (high vs. low functioning). This definition was based on the available Katz scale for residents before day of disease onset. The Katz scale is a measure of independent activity of daily living.
Data processing and quality control
Anonymized data were imported in a relational database for processing, using Extract, Transform, and Load (ETL) techniques. All received anonymized data were then evaluated on basic data quality attributes such as completeness (i.e., the extent of missing data) and accuracy (i.e., whether or not suspicious outliers were present in the individual attributes). Data were enriched with ATC codes for the included drugs. Suggestive symptoms were searched for, based on biometrical measurements as well as indications in text. For the later, basic Natural Language Processing (NLP) techniques were used. For the residents still in the hospital on the moment of data extraction, median imputation was used to estimate length of hospital duration. Two independent physicians manually verified all recorded symptoms as well as all data for random subsample.
Statistical Analysis
We calculated the distributions for dependent and independent variables for the total cohort using appropriate measures of central tendency and dispersion. For our main analysis, we investigated the association between ACEi/ARB and/or statin treatment and 1) serious disease, measured as long-stay hospital admission or death, or 2) asymptomatic disease using a series of logistic regressions applying Firth’s correction. This procedure has been used previously by our group and shown to be robust for low prevalence events and low-dimensional settings (16, 22, 23). We first explored the independent association between ACEi/ARB and both outcomes, as well as the association between statins and the same outcomes. Then we adjusted the models stepwise for age, sex, functional status, hypertension, and diabetes mellitus. All statistical analyses were performed using SAS 9.4 (SAS Institute, North Carolina, United States) and RStudio 3.5.2 (R Foundation for Statistical Computing, Vienna, Austria).
Results
The study cohort included 154 COVID-19-diagnosed residents aged 86±7 (mean±SD) years, evenly distributed over the two nursing homes (76 and 78 residents, respectively). Baseline characteristics are shown in Table 1. In our cohort (33% male), 20% were taking ACEis/ARBs (16% ACEi and 4% ARB), and 20% were taking a statin. Eight residents (5%) were taking both an ACEi/ARB and a statin. Important, none of the residents stopped ACEi/ARB or statin treatment on the day of disease onset and all continued taking their drugs during the follow-up period unless the clinical situation no longer allowed this. Also, none of the residents was taking other renin-angiotensin system (RAS)-associated drugs such as renin-inhibitors or neprylisine-inhibitors. Clinical symptoms detected by NLP in unstructured texts were all manually verified, with 22% false positives, mostly due to mentioned symptoms with more complex negations in the same sentence. Two physicians also independently evaluated manually all available data from a minimum of five random residents each. This resulted in no changes in the result-matrix.
Of the 154 residents, 41 remained asymptomatic during the study period, i.e. 27% of the total cohort and 47% of the PCR-tested COVID-19+ residents. These numbers are similar to those from another study in a similar population (24). Thirty-seven residents (24%) experienced serious COVID-19. Although this serious outcome number seems high compared to other outpatient population studies, in view of the very vulnerable population this is not surprising (25, 26). Among residents treated with ACEis or ARBs, 10/30 (33%) remained asymptomatic vs. 31/124 (25%) of those without such treatment. Residents taking statins remained asymptomatic in 45% of the cases (14/31) vs. 22% (27/123) of those not taking statins. Evaluating COVID-19 severity, 20% (6/30) of the residents treated with ACEi/ARB died or were admitted to hospital for long-stay vs. 25% (31/124) of those without such treatment. Residents taking statins experienced serious COVID-19 in 19% of the cases (6/31) vs. 25% (31/123) of those not taking statins. Interestingly, six of eight residents (75%) taking the ACEi/ARB and statin combination remained asymptomatic throughout the study period. Only one of them (13%) experienced serious COVID-19.
Although not reaching statistical significance, findings from unadjusted logistic regression suggested a potential beneficial effect on COVID-19 symptoms among residents taking ACEis or ARBs (OR 1.52; CI 0.62-3.50; p=0.329). Odds ratios adjusted for age, sex, functional status, diabetes and hypertension were of similar magnitude (Table 2). The results for the statins were most interesting, as we observed a clear and statistically significant association between statin intake and asymptomatic status (unadjusted OR 2.91; CI 1.27-6.71; p=0.011). This association was partially attenuated but remained statistically significant when adjusted for gender, age, functional status, diabetes and hypertension (Table 2).
We also examined associations between ACEis/ARBs and statins, and serious COVID-19. Although the available data failed to reach statistical significance, the directionality of the odds ratios suggested a potential beneficial clinical effect of both ACEi/ARB and statins on serious COVID-19 outcome. All odds ratios (unadjusted as well as adjusted for covariates), were between 0.48 (CI 0.10-1.97; p=0.316) and 0.84 (CI 0.27-2.14; p=0.736) (Table 3).
We did not undertake regression analyses on the combined ACEi/ARB+statin group as there were only eight residents in our cohort; nor did we undertake separate analyses for the ACEi or ARB groups; only six residents were treated with an ARB.
Sensitivity analyses were conducted on the statistically significant association between statins and symptoms. We found that estimates of the impact of statin treatment on asymptomatic status were consistently of the same magnitude and statistically significant as the original analyses.
Table 1. Characteristics of the study cohort. All variables are shown as N (% of column), except age which is mean in years (SD). ACEi = Angiotensin converting enzyme inhibitor; ARB = Angiotensin receptor blocker.
Sample characteristics | Total (N = 154) | ACEi/ARB (N = 30) | No ACEi/ARB (N = 124) | Statin (N = 31) | No statin (N = 123) | Symptoms (N = 113) | No symptoms (N = 41) | Serious COVID (N = 37) | Non-serious COVID (N = 117) |
---|---|---|---|---|---|---|---|---|---|
Age | 85.9 (7.2) | 86.2 (6.6) | 85.8 (7.4) | 85.6 (5.3) | 85.9 (7.6) | 86.0 (7.4) | 85.6 (6.6) | 86.8 (6.8) | 85.6 (7.3) |
Male | 51 (33.1%) | 12 (40.0%) | 39 (31.5%) | 10 (32.3%) | 41 (33.3%) | 41 (36.3%) | 10 (24.4%) | 12 (32.4%) | 39 (33.3%) |
On ACEi/ARB | 30 (19.5%) | 30 (100%) | 0 (0%) | 8 (25.8%) | 22 (17.9%) | 20 (17.7%) | 10 (24.4%) | 6 (16.2%) | 18 (20.5%) |
On statin | 31 (20.1%) | 8 (26.7%) | 23 (18.5%) | 31 (100%) | 0 (0%) | 18 (15.9%) | 14 (34.1%) | 6 (16.2%) | 25 (21.4%) |
Low functioning | 137 (89.0%) | 23 (76.7%) | 114 (91.9%) | 26 (83.9%) | 111 (90.2%) | 106 (93.8%) | 31 (75.6%) | 35 (94.6%) | 102 (87.2%) |
Diabetes mellitus | 28 (18.2%) | 6 (20.0%) | 22 (17.8%) | 10 (32.3%) | 18 (14.6%) | 18 (15.9%) | 10 (24.4%) | 7 (18.9%) | 21 (17.9%) |
Hypertension | 39 (25.3%) | 28 (93.3%) | 11 (8.87%) | 8 (25.8%) | 31 (25.2%) | 29 (25.7%) | 10 (24.4%) | 10 (27.0%) | 29 (24.8%) |
Symptoms | 113 (73.4%) | 20 (66.7%) | 93 (75.0%) | 17 (54.8%) | 96 (78.0%) | 113 (100%) | 0 (0%) | 36 (97.3%) | 77 (65.8%) |
Serious COVID | 37 (24.0%) | 6 (20.0%) | 31 (25.0%) | 6 (19.4%) | 31 (25.2%) | 36 (31.9%) | 1 (2.44%) | 37 (100%) | 0 (0%) |
Table 2. Summary of odds ratios for the asymptomatic COVID-19 infection using logistic regression with Firth’s correction.
Drug treatment | Adjustments | OR (95% CI) on drug vs. no drug | P-value |
---|---|---|---|
ACEi/ARB | - | 1.52 (0.62-3.50) | 0.339 |
Age, sex | 1.61 (0.65-3.80) | 0.283 | |
Age, sex, functional status | 1.35 (0.51-3.31) | 0.521 | |
Age, sex, functional status, diabetes mellitus, hypertension | 2.72 (0.59-25.1) | 0.242 | |
Statins | - | 2.91 (1.27-6.71) | 0.011 |
Age, sex | 2.88 (1.26-6.83) | 0.013 | |
Age, sex, functional status | 2.87 (1.23-7.07) | 0.016 | |
Age, sex, functional status, diabetes mellitus, hypertension | 2.65 (1.13-6.68) | 0.028 |
Table 3. Summary of odds ratios for the serious COVID-19 infection using logistic regression with Firth’s correction.
Drug treatment | Adjustments | OR (95% CI) on drug vs. not drug | P-value |
---|---|---|---|
ACEi/ARB | - | 0.79 (0.26-1.95) | 0.629 |
Age, sex | 0.78 (0.25-1.93) | 0.61 | |
Age, sex, functional status | 0.84 (0.27-2.14) | 0.736 | |
Age, sex, functional status, diabetes mellitus, hypertension | 0.48 (0.10-1.97) | 0.316 | |
Statins | - | 0.75 (0.25-1.85) | 0.556 |
Age, sex | 0.75 (0.25-1.86) | 0.564 | |
Age, sex, functional status | 0.77 (0.25-1.91) | 0.597 | |
Age, sex, functional status, diabetes mellitus, hypertension | 0.75 (0.24-1.87) | 0.559 |
Discussion
There are currently no licensed antiviral treatments for COVID-19 approved. Also the development of COVID-19 vaccines will take time. Moreover, there is no information on when sufficient vaccine supplies will become widely available. Recently, the World Health Organization (WHO) communicated a Solidarity “megatrial” evaluating four broad-spectrum antiviral agents. Among them, the broad-spectrum experimental antiviral drug remdesivir was shown to have low efficacy against Ebola and dropped from further study, although a recent report of its compassionate use in serious COVID-19 was favourable (27). Lopinavir and ritonavir (a protease inhibitor combination used to treat HIV patients) were ineffective in a Chinese clinical trial (28). A lot of attention has gone to chloroquine and hydroxychloroquine. Unfortunately prospects for their success against COVID-19 are not good (29). Convalescent sera, obtained from recovered COVID-19 patients, might be an option to treat acute COVID-19 infections (30), but its implementation will be cumbersome and unlikely to become widely available. The first clinical trials of ACEi/ARB and statin treatments in hospital settings have been initiated within the past month. While we await the results of these trials, which are expected in 2021, this retrospective study should be regarded as both timely and complementary, as it has focused on a frail, non-hospitalised population and demonstrated clinical findings on the use of ACEi/ARB/statins using real world data.
Although statistically not significant, overall both ACEi/ARB and statins show clinical beneficial odds ratios for the outcome serious COVID-19 in elderly people who live in nursing homes. The results for statins and symptoms are most convincing, i.e. large effect sizes which are statistically significant. Statins are most frequently used to prevent cardiovascular diseases. The safety profile of statins is well known and excellent, even in the old population. Moreover, these drugs are relatively inexpensive and widespread, some even as food supplements as red yeast rice, making them easily available throughout the world. Although this observational study does not have the power of a randomized controlled clinical trial, in the current absence of other valuable therapies and considering the benefit-risk balance, an older person living in a nursing home could consider taking a statin if at high COVID-19 infection risk. Currently, therapeutic decisions for COVID-19 patients are driven by observational studies (31, 32). In any case, based on our results, we recommend against stopping statins in patients who are COVID-19-infected.
The combination of ACEi/ARB and statin treatment seemed to have additive beneficial effects on symptoms and serious disease outcome: six of eight residents taking the combination remained asymptomatic and only one of them developed serious COVID-19. Although this result is promising, our sample size was too small to allow us to draw firm conclusions.
One strength of this study is the specific population, i.e., old people (mean age > 85years) residing in nursing homes. Although they are considered highly vulnerable to COVID-19 clinical outcomes, no study has yet reported on the effects of ARB/ACEi and/or statin treatment on COVID-19 in this population. Extracting reliable data from nursing homes with COVID-19 outbreaks is far more cumbersome than extracting data from hospitals. Another strength is that drug treatment was based on real intake, in contrast to most hospital-based studies that use prescriptions as proxies for drug treatment. Lastly, in contrast to most hospital studies, asymptomatic COVID-19 patients were included in the study. People admitted to hospitals are evidently always symptomatic.
One limitation of our study is its relatively small cohort size. Consequently, absence of statistical significance should be interpreted with caution. However, the consistency in the observed effect sizes, even without statistical significance due to small sample size, should be considered in the overall evaluation. As number of cases increase, further analyses will be undertaken to better understand our findings and confirm these associations. Also, another limitation was the lack of other potential confounders, including chronic kidney injury and BMI. Finally, our results apply to a very specific population (elderly people living in nursing homes) and cannot be generalized to other groups such as young people or hospitalized people.
Conclusion
Our study, based on available data, indicates that in elderly nursing home residents, statin treatment is associated with beneficial effects on COVID-19-related clinical symptoms. Although not statistically significant, our findings also suggested that statin treatment in combination with an ACEi or ARB was associated with less severe clinical outcomes. In the light of these findings, a prudent recommendation is to continue or initiate statin treatment for older people residing in nursing homes and at high risk for COVID-19 infection.
Acknowledgements
We thank all of the staff of VZW Zorg-Saam Zusters Kindsheid Jesu for their daily care of older people and for their collaboration on this study during these difficult times. We also thank the staff of the Corilus Health IT Center who helped with the data extraction.
References
- Kuba K, Imai Y, Rao SA, Gao H, Guo F, Guan B, et al. A crucial role of angiotensin converting enzyme 2 (ACE2) in SARS coronavirus-induced lung injury. Nature Medicine. 2005;11(8):875-9.
- Imai Y, Kuba K, Rao S, Huan Y, Guo F, Guan B, et al. Angiotensin-converting enzyme 2 protects from severe acute lung failure. Nature. 2005;436(7047):112-6.
- Wosten-van Asperen RM, Bos AP, Bem RA, Dierdorp BS, Dekker T, van Goor H, et al. Imbalance Between Pulmonary Angiotensin-Converting Enzyme and Angiotensin-Converting Enzyme 2 Activity in Acute Respiratory Distress Syndrome. Pediatric Critical Care Medicine. 2013;14(9):E438-E41.
- Woesten-van Asperen RM, Lutter R, Specht PA, Moll GN, van Woensel JB, van der Loos CM, et al. Acute respiratory distress syndrome leads to reduced ratio of ACE/ACE2 activities and is prevented by angiotensin-(1-7) or an angiotensin II receptor antagonist. Journal of Pathology. 2011;225(4):618-27.
- Fedson DS. Treating the host response to emerging virus diseases: lessons learned from sepsis, pneumonia, influenza and Ebola. Annals of Translational Medicine. 2016;4(21).
- McGonagle D, Sharif K, O’Regan A, Bridgewood C. The Role of Cytokines including Interleukin-6 in COVID-19 induced Pneumonia and Macrophage Activation Syndrome-Like Disease. Autoimmunity reviews. 2020:102537.
- O’donnell J, Sharif K, Emery P, Bridgewood C, Mcgonagle D. Why the Immune Mechanisms of Pulmonary Intravascular Coagulopathy in COVID-19 Pneumonia are Distinct from Macrophage Activation Syndrome with Disseminated Intravascular Coagulation. DOI: 10.13140/RG.2.2.19782.83521; 2020.
- Tang N, Li D, Wang X, Sun Z. Abnormal coagulation parameters are associated with poor prognosis in patients with novel coronavirus pneumonia. Journal of Thrombosis and Haemostasis. 2020;18(4):844-7.
- Yin S, Huang M, Li D, Tang N. Difference of coagulation features between severe pneumonia induced by SARS-CoV2 and non-SARS-CoV2. Journal of Thrombosis and Thrombolysis. 2020.
- van de Veerdonk F, Netea MG, van Deuren M, van der Meer JW, de Mast Q, Bruggemann RJ, et al. Kinins and Cytokines in COVID-19: A Comprehensive Pathophysiological Approach. Doi: 1020944/preprints2020040023v1. 2020
- Schol-Gelok S, Morelli F, Arends LR, Boersma E, Kruip MJHA, Versmissen J, et al. A revised systematic review and meta-analysis on the effect of statins on D-dimer levels. European Journal of Clinical Investigation. 2019;49(8).
- Aktas S, Ucak S, Kurt F, Tasdemir M, Kutlu O, Eker P. Evaluation of protein C and protein S levels in patients with diabetes mellitus receiving therapy with statins and ACE inhibitors or angiotensin II receptor blockers. Diabetes Research and Clinical Practice. 2018;135:88-92.
- Mortensen E, Anzueto A. Prior use of both a statin and ARB is associated with lower mortality for patients hospitalized with pneumonia. European Respiratory Journal. 2016;48.
- Mortensen EM, Nakashima B, Cornell J, Copeland LA, Pugh MJ, Anzueto A, et al. Population-Based Study of Statins, Angiotensin II Receptor Blockers, and Angiotensin-Converting Enzyme Inhibitors on Pneumonia-Related Outcomes. Clinical Infectious Diseases. 2012;55(11):1466-73.
- Meng J, Xiao G, Zhang J, He X, Ou M, Bi J, et al. Renin-angiotensin system inhibitors improve the clinical outcomes of COVID-19 patients with hypertension. Emerging Microbes & Infections. 344 2020;9(1):757-60.
- Bean D, Kraljevic Z, Searle T, Bendayan R, Pickles A, Folarin A, et al. Treatment with ACE-inhibitors is associated with less severe SARS-Covid-19 infection in a multi-site UK acute Hospital Trust. Doi: 1013140/RG223488314889/1.2020.
- Guang Y, Zihu T, Ling Z, Min Y, Lang P, Jinjin L, et al. Angiotensin II Receptor Blockers and Angiotensin-Converting Enzyme Inhibitors Usage is Associated with Improved Inflammatory Status and Clinical Outcomes in COVID-19 Patients With Hypertension. Doi: 101101/2020033120038935. 2020.
- Yingxia L, Fengming H, Jun X, Penghui Y, Yuhao Q, Mengli C, et al. Anti-hypertensive Angiotensin II receptor blockers associated to mitigation of disease severity in elderly COVID-19 353 patients. Doi: 10.1101/2020.03.20.20039586; 2020.
- Zhang P, Zhu L, Cai J, Lei F, Qin J-J, Xie J, et al. Association of Inpatient Use of Angiotensin Converting Enzyme Inhibitors and Angiotensin II Receptor Blockers with Mortality Among Patients With Hypertension Hospitalized With COVID-19. Circulation research. 2020.
- https://covid19.sciensano.be/nl/covid-19-epidemiologische-situatie.
- https://www.businessinsider.com/us-coronavirus-deaths-at-nursing-homes-2020.
- Zhang QY. Firth logistic regression for rare variant association tests. Frontiers in Genetics. 360 2014;5.
- Firth D. BIAS REDUCTION OF MAXIMUM-LIKELIHOOD-ESTIMATES. Biometrika. 1993;80(1):27-38.
- McMichael TM, Currie DW, Clark S, Pogosjans S, Kay M, Schwartz NG, et al. Epidemiology of Covid-19 in a Long-Term Care Facility in King County, Washington. The New England journal of medicine. 2020.
- Onder G, Rezza G, Brusaferro S. Case-Fatality Rate and Characteristics of Patients Dying in Relation to COVID-19 in Italy. Jama. 2020.
- O’Dowd A. Covid-19: Deaths in care home deaths in England and Wales rise sharply. BMJ (Clinical research ed). 2020;369:m1727-m.
- Grein J, Ohmagari N, Shin D, Diaz G, Asperges E, Castagna A, et al. Compassionate Use of Remdesivir for Patients with Severe Covid-19. The New England journal of medicine. 2020.
- Cao B, Wang Y, Wen D, Liu W, Wang J, Fan G, et al. A Trial of Lopinavir-Ritonavir in Adults Hospitalized with Severe Covid-19. The New England journal of medicine. 2020.
- Cortegiani A, Ingoglia G, Ippolito M, Giarratano A, Einav S. A systematic review on the efficacy and safety of chloroquine for the treatment of COVID-19. Journal of critical care. 2020.
- Casadevall A, Pirofski L-A. The convalescent sera option for containing COVID-19. The Journal of clinical investigation. 2020;130(4):1545-8.
- Paranjpe I, Fuster V, Lala A, Russak A, Glicksberg BS, Levin MA, et al. Association of Treatment Dose Anticoagulation with In-Hospital Survival Among Hospitalized Patients with COVID-19. J Am Coll Cardiol. 2020;May 06:Epublished.
- https://www.tctmd.com/news/anticoagulants-may-aid-covid-19-patients-nyc-data-suggest.
Risk prediction for poor outcome and death in hospital in-patients with COVID-19: derivation in Wuhan, China and external validation in London, UK
First online: 03-05-2020 | Last update: 04-05-2020
Authors
Huayu Zhang, MSc*1, Ting Shi, PhD*2, Xiaodong Wu, MD*3,4, Xin Zhang, MD5, Kun Wang, MD3,6, Daniel Bean, PhD7,8, Richard Dobson, PhD7,8,9, James T Teo, MD10, Jiaxing Sun, MD3, Pei Zhao, MD3, Chenghong Li, MD11, Kevin Dhaliwal, PhD12, Honghan Wu, PhD†1,13,14, Qiang Li, MD†3, Bruce Guthrie, PhD†15
*Contributed equally †Joint corresponding authors
Affiliations
- Centre for Medical Informatics, Usher Institute, University of Edinburgh, Scotland, United Kingdom
- Centre for Global Health, Usher Institute, University of Edinburgh, Scotland, United Kingdom
- Department of Pulmonary and Critical Care Medicine, Shanghai East Hospital, Tongji University, Shanghai, China
- Department of Pulmonary and Critical Care Medicine, Taikang Tongji Hospital, Wuhan, China
- Department of Pulmonary and Critical Care Medicine, People’s Liberation Army Joint Logistic Support Force 920th Hospital, Yunnan, China
- Department of Pulmonary and Critical Care Medicine, Shanghai General Hospital, Shanghai Jiaotong University School of Medicine, Shanghai, China
- Department of Biostatistics and Health Informatics, Institute of Psychiatry, Psychology and Neuroscience, King’s College London, London, England, United Kingdom
- Health Data Research UK London, University College London, London, UK
- Institute of Health Informatics, University College London, London, England, United Kingdom
- Department of Stroke and Neurology, King’s College Hospital NHS Foundation Trust, London, England, United Kingdom
- Department of Pulmonary and Critical Care Medicine, Wuhan Sixth Hospital, Jianghan
University, Wuhan, China - Centre for Inflammation Research, Queens Medical Research Institute, University of Edinburgh, Scotland, United Kingdom
- School of Computer and Software, Nanjing University of Information Science and Technology, Nanjing, China
- Health Data Research UK London, University of Edinburgh, London, UK
- Centre for Population Health Sciences, Usher Institute, University of Edinburgh, Scotland, United Kingdom
Lead corresponding author
Bruce Guthrie, Professor of General Practice, University of Edinburgh UK
e-mail bruce.guthrie@ed.ac.uk
Tel +44 7948 267 273
Word count 3492
Citation
Zhang, Huayu, et al. “Risk prediction for poor outcome and death in hospital in-patients with COVID-19: derivation in Wuhan, China and external validation in London, UK.” medRxiv 2020.04.28.20082222. https://doi.org/10.1101/2020.04.28.20082222
Research in context
Evidence before this study
Several prognostic models for predicting mortality risk, progression to severe disease, or length of hospital stay in COVID-19 have been published.1 Commonly reported predictors of severe prognosis in patients with COVID-19 include age, sex, computed tomography scan features, C-reactive protein (CRP), lactic dehydrogenase, and lymphocyte count. Symptoms (notably dyspnoea) and comorbidities (e.g. chronic lung disease, cardiovascular disease and hypertension) are also reported to have associations with poor prognosis.2 However, most studies have not described the study population or intended use of prediction models, and external validation is rare and to date done using datasets originating from different Wuhan hospitals.3 Given different patterns of testing and organisation of healthcare pathways, external validation in datasets from other countries is required.
Added value of this study
This study used data from Wuhan, China to derive and internally validate multivariable models to predict poor outcome and death in COVID-19 patients after hospital admission, with external validation using data from King’s College Hospital, London, UK. Mortality and poor outcome occurred in 4.3% and 9.7% of patients in Wuhan, compared to 34.1% and 42.9% of patients in London. Models based on age, sex and simple routinely available laboratory tests (lymphocyte count, neutrophil count, platelet count, CRP and creatinine) had good discrimination and calibration in internal validation, but performed only moderately well in external validation. Models based on age, sex, symptoms and comorbidity were adequate in internal validation for poor outcome (ICU admission or death) but had poor performance for death alone.
Implications of all the available evidence
This study and others find that relatively simple risk prediction models using demographic, clinical and laboratory data perform well in internal validation but at best moderately in external validation, either because derivation and external validation populations are small (Xie et al3) and/or because they vary greatly in casemix and severity (our study). There are three decision points where risk prediction may be most useful: (1) deciding who to test; (2) deciding which patients in the community are at high-risk of poor outcomes; and (3) identifying patients at high-risk at the point of hospital admission. Larger studies focusing on particular decision points, with rapid external validation in multiple datasets are needed. A key gap is risk prediction tools for use in community triage (decisions to admit, or to keep at home with varying intensities of follow-up including telemonitoring) or in low income settings where laboratory tests may not be routinely available at the point of decision-making. This requires systematic data collection in community and low-income settings to derive and evaluate appropriate models.
Abstract
Background
Accurate risk prediction of clinical outcome would usefully inform clinical decisions and intervention targeting in COVID-19. The aim of this study was to derive and validate risk prediction models for poor outcome and death in adult inpatients with COVID-19.
Methods
Model derivation using data from Wuhan, China used logistic regression with death and poor outcome (death or severe disease) as outcomes. Predictors were demographic, comorbidity, symptom and laboratory test variables. The best performing models were externally validated in data from London, UK.
Findings
4.3 % of the derivation cohort (n=775) died and 9.7% had a poor outcome, compared to 34.1% and 42.9% of the validation cohort (n=226). In derivation, prediction models based on age, sex, neutrophil count, lymphocyte count, platelet count, C-reactive protein and creatinine had excellent discrimination (death c- index=0.91, poor outcome c-index=0.88), with good-to-excellent calibration. Using two cut-offs to define low, high and very-high risk groups, derivation patients were stratified in groups with observed death rates of 0.34%, 15.0% and 28.3% and poor outcome rates 0.63%, 8.9% and 58.5%. External validation discrimination was good (c-index death=0.74, poor outcome=0.72) as was calibration. However, observed rates of death were 16.5%, 42.9% and 58.4% and poor outcome 26.3%, 28.4% and 64.8% in predicted low, high and very-high risk groups.
Interpretation
Our prediction model using demography and routinely-available laboratory tests performed very well in internal validation in the lower-risk derivation population, but less well in the much higher-risk external validation population. Further external validation is needed. Collaboration to create larger derivation datasets, and to rapidly externally validate all proposed prediction models in a range of populations is needed, before routine implementation of any risk prediction tool in clinical care.
Funding
MRC, Wellcome Trust, HDR-UK, LifeArc, participating hospitals, NNSFC, National Key R&D Program, Pudong Health and Family Planning Commission
Introduction
Severe acute respiratory syndrome coronavirus-2 (SARS-CoV-2) is the novel coronavirus that causes coronavirus disease 2019 (COVID-19). A local outbreak of COVID-19 was first detected in Wuhan, China and rapidly spread to cause a pandemic.4
A key feature of the pandemic in all areas with large local epidemics is that healthcare systems are often rapidly overwhelmed. Effective and safe triage to ensure that those at highest risk of severe disease are appropriately escalated, and those at lower risk safely monitored, is critical to maintain healthcare system capacity for as long as possible. The same risk stratification is also needed to support targeted recruitment to both mechanistic and interventional research studies, since developing effective treatments of early disease is a key objective alongside vaccine development. Although risk stratification is important in all health systems, exactly how risk stratification is done will vary by country and by healthcare system. Across all systems though, important care pathway decision points include (1) who to test (particularly important when tests are in short supply); (2) who to hospitalize, and who to care for in the community (with or without telemonitoring or other proactive follow-up), and; (3) who to direct down different care pathways after admission. Since the severity of disease and risk of poor outcome varies by point on the pathway, there is a need for a range of risk stratification tools based on the data available at different stages of the care pathway (Figure 1), and/or in different healthcare systems.
A number of studies have identified patient characteristics associated with poor outcomes in COVID-19 using univariate analysis. Older age, being male, dyspnea and comorbidities (e.g. chronic obstructive airways disease [COPD], cardiovascular disease, hypertension) are associated with intensive care unit (ICU) admission,2,5-11 and comorbidities are also reported to be associated with an increased risk of death.12,13 However, many studies do not report adjusted associations and few examine laboratory findings, although there are reported univariate associations between the development of acute respiratory distress syndrome (ARDS) and a range of predictors including high fever (≥39°C), older age, and laboratory measures including neutrophilia, d-dimer and other evidence of coagulation and organ dysfunction.14 However, univariate analysis is usually a poor guide to risk because of confounding, most obviously between age and physical morbidities.15
Two studies have published multivariable/adjusted findings. Zhou et al examined risk factors for in- hospital death in 191 patients, finding significant adjusted associations with older age, higher Sequential Organ Failure Assessment (SOFA) score, lower lymphocyte count and increased d-dimer.16 Xie et al is the only published study explicitly aiming to create a risk prediction model for inpatient mortality for clinical use, with derivation in 299 patients admitted to one Wuhan hospital and external validation in 145 patients admitted to another Wuhan hospital.3 Mortality was very high in both derivation (51.8%) and validation (47.6%) cohorts. Factors associated with inpatient mortality were age, lower lymphocyte count, higher lactate dehydrogenase and lower peripheral capillary oxygen saturation (SpO2), and external validation showed reasonable performance (calibration was excellent but only after model recalibration to the validation dataset which will rarely be possible in clinical use).3 Neither study reported clinically relevant performance measures such as sensitivity, specificity and positive/negative predictive value.
The aim of this study was to derive and internally validate risk prediction models for poor outcome and death in a cohort of 775 inpatients with COVID-19 in Wuhan, China, with external validation in a cohort of 226 inpatients with COVID-19 in London, UK.
Methods
Methods are briefly described here and in detail in the supplementary file.
Study design and participants
The multivariable risk prediction model was derived in a cohort of 775 adults with COVID-19 confirmed by RT-PCR admitted to one of two hospitals in Wuhan, China (Wuhan Sixth Hospital and Taikang Tongji Hospital). The model was externally validated in a cohort of 226 adult inpatients with confirmed COVID-19 in King’s College Hospital (KCH) NHS Foundation Trust, London, UK.
Statistical modelling
A logistic regression model was chosen for its good clinical interpretability. Models were fitted with L1- regularization (LASSO) to minimize overfitting. Two clinically relevant outcomes were defined: death and poor outcome (defined as: developing ARDS, receiving intubation or extracorporeal membrane oxygenation (ECMO) treatment, ICU admission and death). Predictor variables were participants’ demographic characteristics, premorbid conditions, symptoms and laboratory test results (around admission) as predictors. Combinations of predictors were made with consideration of information availability at points of the health care pathway (Figure 1):
- Community triage: Demographic + premorbid Conditions + Symptoms (DCS)
- Hospital admission (full model): DCS + Laboratory results (DCSL)
- Hospital admission (simpler model): Demographic + Laboratory results (DL)
Models are referred to as predictor-outcome (e.g. DCS-Death). For each model, predictors with coefficients significantly different to zero in cross-validation were selected. Optimal L1-regularization strength for preferred models was chosen by best C-index in cross-validation. All models were internally validated with cross-validation to select variables based on model fit and not just individual predictor statistical significance, and model performance metrics in the held-out partition were reported. To explore prediction cut-offs to use to inform clinical decision-making, we grouped patients into low-, high- and very high-risk groups based on predicted probabilities obtained from the final models. Cut-offs to define the low and high-risk groups were arbitrarily assigned to be approximately 10-fold lower and 8-fold higher than the outcome percentage in the whole dataset. Models using DL predictors were externally validated in the KCH cohort, using two outcomes: death and poor outcome (defined as ICU admission or death).
Ethics and governance
The derivation study was approved by the Research Ethics Committee of Shanghai Dongfang Hospital and Taikang Tongji Hospital. The external validation study operated under London South East Research Ethics Committee (reference 18/LO/2048) approval granted to the King’s Electronic Records Research Interface (KERRI).
Results
Description of the patient cohort
The derivation cohort consisted of 775 adult in-patients with COVID-19 in Wuhan (Table 1). The median age was 61 years (IQR 50-68, range 18-96), 48.9% were men, and 345 (44.5%) had comorbidity (most commonly hypertension (30.8%), diabetes mellitus (13.7%) and heart disease (11.0%)). The most common symptoms on admission were fever (68.6%) and cough (68.1%), followed by fatigue (54.3%) and dyspnoea (45.8%). Median lymphocyte count was 1.4 (IQR 1.1-1.9) 10^9/L, neutrophil count 3.5 (2.7-4.9) 10^9/L, platelet count 224 (180-274) 10^9/L, CRP 2.7 (0.9-14.1) mg/L and creatinine 63.9 (54.1-76.4) µmol/L.
The validation cohort consisted of 226 adult patients admitted to Kings College Hospital, London with a positive COVID-19 RT-PCR, and with complete data for the laboratory tests included in the derivation risk prediction model (Table 1). Median age was 74 years (IQR 59-85, range 19-98) and 54.9% were men. Data on symptoms and comorbidity were not extracted. Median lymphocyte count was 1.1 (IQR 0.7-1.6) 10^9/L, neutrophil count 4.9 (3.4-6.9) 10^9/L, platelet count 211 (160-284) 10^9/L, CRP 56.0 (21.1-115.5) mg/L, and creatinine 90.0 (68.0-126.0 µmol/L.
Median lymphocyte count and platelet count was lower, and median age, proportion male, neutrophil count, CRP and creatinine higher in the KCH validation compared to the Wuhan derivation cohort. In the derivation cohort, 33 (4.3%) died and 75 (9.7%) had pre-defined poor outcome (ARDS, intubation, ECMO, ICU admission or death) (two patients had missing data for death and three patients had missing data for poor outcome). Both outcomes were much more common in the validation cohort where 77 (34.1%) died, and 97 (42.9%) of patients experienced a poor outcome (ICU admission or death).
Univariate associations of patient characteristics and outcomes are summarised in Table S1, with findings broadly consistent with previously reported univariate associations.2,12,13 The strongest observed univariate associations were increased risk of each outcome with increasing age, the presence of prior comorbidity (particularly chronic lung disease, heart disease, immunocompromise, chronic renal disease and hypertension), the presence of dyspnoea, and laboratory values (increased risk with higher neutrophil count, CRP and creatinine, and with lower lymphocyte count and platelet count).
Derivation of prediction models in the Wuhan cohort
Table 2 summarises multivariable odds ratios of models for both outcomes (Table S2 reports the predictor coefficients with more precision), and Table 3 details model performance in internal and external validation. In adjusted analysis, underlying conditions and symptoms were no longer associated with either outcome with the exception of dyspnoea which was associate with poor outcome, although confidence intervals are wide meaning that a type 2 error cannot be ruled out.
For the outcome of death, DCS-Death, DCSL-Death and DL-Death models achieved C-indices of 0.79, 0.89 and 0.91, respectively (Table 3 and Figure S1A). Calibration evaluated using calibration-in-the-large and calibration slope was poor for DCS-Death and DCSL-Death models, but good for DL-Death (calibration-in-the-large 0.19 [perfect calibration=0], calibration slope 0.98 [perfect calibration=1]).
In the DL-Death model, increased blood neutrophil counts (OR 1.25 [all OR for continuous variables are per one unit increase in predictor values], p=0.002) and CRP levels (OR 1.01, p=0.008) were significantly associated with death. Other variables were not statistically significantly associated with death but included because they improved model fit.
Using a probability of 0.5 as the prediction cut-off for the DL-Death model, sensitivity was 0.33, positive predictive value 0.52, specificity 0.99, and negative predictive value 0.98, which is not adequate for clinical use. Using the two alternative probability cut-offs to define low, high, and very high risk groups, the percentage who died in the low, high, and very high risk groups were 0.34% (n=2/580), 15.0% (n=3/20) and 28.3% (n=15/53), respectively (Figure 2A-B, Table S3). Notably, the model defined a low- risk group comprising 88.8% of patients with very high negative predictive value (0.997) (Table 3) as well as a very-high risk group comprising 8.1% of patients in whom 75% of observed deaths occurred.
For poor outcome, the DCS-Poor, DCSL-Poor and DL-Poor models achieved C-indices of 0.80, 0.88 and 0.88, respectively (Table 3, Figure S1B). Calibration was better than for models predicting death, and was very good for the DL-Poor model (calibration-in-the-large 0.01, calibration slope 1.04).
In the DL-Poor model, risk of poor outcome of COVID-19 was associated with increased blood neutrophil counts (OR 1.29 [all OR for continuous variables are per one unit increase in predictor value], p=<0.001), increased creatinine levels (OR 1.01, p=0.002) and decreased blood lymphocyte counts (OR 0.31, p=0.004). Age (OR 1.03, p=0.053) and CRP (OR 1.01, p=0.060) were strongly associated but marginally not statistically significant in adjusted models. Other variables were not statistically significantly associated with death but included because they improved model fit.
Using a probability of 0.5 as the prediction cut-off for the DL-Poor model, sensitivity was 0.36, positive predictive value 0.72, specificity 0.99, and negative predictive value 0.94, which is not ideal for clinical use. Using the two alternative probability cut-offs, the percentage of patients with poor outcome in the low, high, and very high-risk groups were 0.63% (n=2/320), 8.9% (n=25/280) and 58.5% (n=31/53), respectively (Figure 2E-F). Notably, the model defined a low-risk group comprising 49.0% of patients with very high negative predictive value (0.99) (Table 3) as well as a very-high risk group comprising 8.1% of patients with high positive predictive value (0.60) in which 53% of observed poor outcomes occurred.
External validation of the model in KCH cohort
Table 3 shows the performance (discrimination, calibration and clinical usefulness) of the DL-Death and DL-Poor models without any re-calibration. Both models showed good discrimination measured by the C-index (death: 0.74; poor outcome: 0.72), but calibration was only fair with calibration-in-the-large 0.25 for death and 0.28 for poor outcome, and calibration slope 0.54 for death and 0.54 for poor outcome. Calibration plots are shown in Figure S2, and show some under-prediction at low levels of risk. Using the default probability cut-off of 0.5, sensitivity was poor (0.23 for death; 0.40 for poor outcome), specificity good to excellent (0.95; 0.85), PPV (0.69; 0.67) and NPV (0.71; 0.65) were fair, given outcome prevalence.
Using the derivation model probability cut-offs for low, high and very high risk groups, the DL-Death model categorized the external validation cohort into groups with death rates of 16.5% (n=20/121), 42.9% (n=12/28) and 58.4% (n=45/77). Sensitivity at the low-high risk cut-off was 0.77, specificity 0.68, PPV 0.55 and NPV 0.85, and at the high-very high risk cut-off sensitivity 0.58, specificity 0.78, PPV 0.58 and NPV 0.78.
Using the derivation model probability cut-offs for low, high and very high risk groups, the DL-Poor model categorized the KCH cohort into groups with outcome rates of 26.3% (n=5/19), 28.4% (n=33/116) and 64.8% (n=59/91). Sensitivity at the low-high risk cut-off was 0.95, specificity 0.11, PPV 0.44 and NPV 0.74, and at the high-very high risk cut-off sensitivity 0.61, specificity 0.75, PPV 0.72 and NPV 0.65.
Discussion
We derived and internally validated models to predict the risk of death and poor outcome for COVID-19 patients using a cohort of inpatients in Wuhan, China with a relatively low risk of death (4.3%) and poor outcome (9.7%). Four groups of predictors were examined: demographic information, premorbid conditions, symptoms and laboratory results. Models including demographic and laboratory data (DL models) had the best performance, with older age, higher neutrophil count, lower lymphocyte count, lower platelet count, higher CRP and higher creatinine being predictive of death and poor outcome. In internal validation, DL model performance was good, and it was possible to define low, high and very- high risk groups for both outcomes. DL models were externally validated in a cohort of inpatients in London, UK with eight-fold higher risk of death (34.1%) and four-fold higher risk of poor outcome (42.9%) reflecting different care pathways in the two countries (Figure 1) and therefore different casemix of those admitted (Table 1). In this very different cohort, DL model performance was only fair to moderate in the external validation dataset, and further external validation is required in datasets with different casemix and severity.
Our study has several strengths. First, model derivation used a relatively large cohort of inpatients with COVID-19 from Wuhan, China with 99.6% ascertainment of endpoints, and predictors measured on admission. Derivation and internal validation of prediction models was robust. Second, we externally validated parsimonious risk prediction models using a dataset from a different country with a very different pattern of COVID-19 admission (London, UK). Third, the study is reported in accordance with the TRIPOD guidelines17 including detailed methods reporting in the supplementary file to facilitate reproducibility and further external validation.1
The study also has a number of limitations. First, the clinical datasets were collected when healthcare services were under severe strain. Data extraction sought to ensure consistency and accuracy, but was not blind to outcome, and there is missing data in both datasets. Second, the datasets used are smaller than ideal (although as large as or larger than previous studies), and there are relatively few deaths in particular. Our analytical approach aimed to minimise overfitting, but further research using larger, federated datasets is clearly required. Third, clinical assessments at admission such as SpO2 are likely to be important predictors of short-term outcome,3 but were not available in either dataset. Fourth, our external validation dataset has very different case-mix where spectrum effects are likely to contribute to lower prediction model performance, and only has follow-up to a fixed date (period range: [6-39] days, although this is a reasonable time-horizon to inform clinical decision-making at hospital admission). Finally, all data available is for people with PCR-diagnosed COVID-19 who are admitted to hospital (decision 3 in Figure 1). Although the Wuhan cohort includes many people with less severe disease, in the validation cohort most admitted patients are likely to have severe disease. The findings therefore cannot be assumed to be applicable to decisions made earlier in the course of disease (decision 2 in Figure 1).
Our univariate findings are similar to other studies reporting univariate associations. Older patients and those with dyspnoea or premorbid conditions (e.g. chronic lung disease, hypertension, cardiovascular disease) are consistently reported to be more susceptible to in-hospital death or poor outcome of COVID-19.2,6,8,9,12,13,18 Lymphocytopenia, neutrophilia, and markers of inflammation (e.g. CRP), cell death (e.g. lactate dehydrogenase (LDH)) and abnormal coagulation (e.g. d-dimer) have all also been reported as associated with poor outcome.14 However, the majority of these studies are small and only reported univariate associations.
Our findings are similar to the few studies reporting multivariable associations, although the predictors included are not identical.3,16 Age is a very strong predictor of outcome, and accounting for age reduces associations with comorbidity and symptoms (although no study including our own is large enough to rule out significant type 2 error i.e. that these are truly not independently associated with outcomes). Lymphocyte count is included in all three models, but Zhou et al also included d-dimer and SOFA score, whereas Xie et al included LDH and SpO2, compared to our inclusion of neutrophil count and CRP. The three models are therefore based on demography, (different) laboratory measures, and (different) clinical measures. The choice of predictors in all three studies is driven by data availability, and by initial univariate analysis identifying different features to model (SOFA score for example is a good predictor in Zhou et al,16 but not in Xie et al’s study.3 There is a need for larger studies to derive and validate more optimal models suitable for use at different points in the natural history of COVID-19, since for example, comorbidity may (or may not) be an important predictor of admission but less important than laboratory markers for predicting prognosis in those admitted.
Xie et al’s prediction model was externally validated in a similar dataset from Wuhan with very high mortality in both datasets (derivation cohort 51.8% died, validation cohort 47.6%). Discrimination in external validation was excellent, but calibration was poor until the model intercept and slope were recalibrated to the external validation dataset. We chose not to recalibrate our model to the external dataset, since this will hardly ever be feasible in a tool intended for clinical use for an epidemic disease (and Xie et al’s nomogram intended for use by clinicians is also based on the derivation model). Xie et al did not publish clinically relevant performance metrics such as sensitivity, specificity, PPV and NPV, making it difficult to evaluate clinical utility.
The findings of this and previous studies are consistent with demography and routinely available laboratory measurements being useful markers of prognosis at the time of hospital admission, indicating that simple risk prediction tools may usefully inform clinical decision-making at this point in the care pathway. However, COVID-19 risk prediction models need external validation before widespread clinical use, because severity and prognosis depends on the setting and system of care. Our Wuhan derivation cohort is closer to a general population cohort (mortality 4.3%) than either our validation cohort in London (mortality 34.1%) or the Wuhan cohorts in other studies (mortality 28.3%,16 and 51.8% derivation and 47.6% validation3). It may therefore be better suited to decisions about who to admit and who can safely be sent home to self-isolate (decision 2 in Figure 1) than to identifying those at highest risk of poor outcome and death in people with more severe disease, for example at the point of potential ICU admission. All proposed prediction models therefore require repeated external validation in multiple datasets to identify the contexts in which they can be most effectively deployed. The DCS and DL models are available as prediction tools in a web portal but we emphasise that they should be used with caution, particularly in settings with different rates of poor outcomes, and are not a replacement for clinical judgement (https://covid.datahelps.life/prediction/).
Although very challenging under epidemic conditions, systematic data collection during clinical care and rapid data analysis is therefore essential to understand the natural history of COVID-19 to inform clinical decision-making along the entire care pathway (Figure 1). Of note is that our models based solely on demography, symptoms and premorbid conditions did not perform optimally in internal validation, which may indicate that community assessment would optimally include simple blood tests. Further research is needed to examine this, and the relative value of laboratory tests compared to clinical examination (e.g. pulse, respiratory rate, SpO2). Key gaps are in data collection and analysis in early disease and in the community (since minimising admission when it is safe to do so is critical for keeping hospital services functioning) and at the point of ICU admission (since there may or may not be populations where mechanical ventilation is predictably futile).
The observed associations between raised neutrophil19 and low lymphocyte counts20 with outcomes highlights that understanding and delineating the innate and adaptive immune cascade may inform potential interventional strategies. These include targeting viral replication alongside directed anti- inflammatory agents to mitigate the inflammatory cascade.21 The timing of such interventions is likely to be crucial, and risk prediction models therefore have the additional potential to support targeted and timely treatment initiation.
Conclusion
Risk prediction tools have considerable potential to inform clinical decision-making at different points in the COVID-19 care pathway. Existing models, including our own, perform well in derivation datasets but robust and repeated external validation is required before widespread clinical use. Collaborative research to derive and validate tools using larger datasets from all points on the clinical pathway is urgently needed.
Author contribution
QL, HW, BG, HZ, TS, and XW conceived the study. All data from the Wuhan cohort were extracted by XZ, JS, PZ, and CL, double-checked by KW and XW, with disagreements resolved by involving a third independent reviewer (QL). Data from the KCH cohort were extracted by DB and reviewed by JTT. HZ, TS, BG, KD and HW analysed the data, with HZ, TS, BG, KD, HW, XW, RD and JTT leading interpretation of findings. All authors participated in the preparation and approval of the Article.
Declaration of interests
We declare no competing interests.
Acknowledgements
HW and HZ are supported by Medical Research Council and Health Data Research UK Grant (MR/S004149/1), Industrial Strategy Challenge Grant (MC_PC_18029) and Wellcome Institutional Translation Partnership Award (PIII054). RD is supported by the National Institute for Health Research (NIHR) Biomedical Research Centre at South London and Maudsley NHS Foundation Trust and King’s College London. DMB is funded by a UKRI Innovation Fellowship as part of Health Data Research UK MR/S00310X/1 (https://www.hdruk.ac.uk). KD is supported by LifeArc STOPCOVID award. This work uses data provided by patients and collected by the NHS as part of their care and support. XW is supported by National Natural Science Foundation of China (grant number:81700006). QL is supported by National Key R&D Program (2018YFC1313700), National Natural Science Foundation of China (grant number: 81870064) and the “Gaoyuan” project of Pudong Health and Family Planning Commission (PWYgy2018-06). The views expressed are those of the authors and not necessarily those of the NHS, the NIHR, or the Department of Health and Social Care. We acknowledge Lingyu Ran and Yongsheng Du for their contribution in data collection.
References
- Wynants L, Van Calster B, Bonten MMJ, et al. Prediction models for diagnosis and prognosis of covid-19 infection: systematic review and critical appraisal. BMJ 2020; 369: m1328.
- Jain V, Yuan J-M. Systematic review and meta-analysis of predictive symptoms and comorbidities for severe COVID-19 infection. medRxiv 2020: 2020.03.15.20035360.
- Xie J, Hungerford D, Chen H, et al. Development and external validation of a prognostic multivariable model on admission for hospitalized patients with COVID-19. medRxiv 2020: 2020.03.28.20045997.
- University JH. Johns Hopkins Coronavirus Resource Center. 2020 (accessed 25 April 2020).
- Li K, Wu J, Wu F, et al. The Clinical and Chest CT Features Associated with Severe and Critical COVID-19 Pneumonia. Invest Radiol 2020.
- Huang C, Wang Y, Li X, et al. Clinical features of patients infected with 2019 novel coronavirus in Wuhan, China. Lancet 2020; 395(10223): 497-506.
- Guan WJ, Ni ZY, Hu Y, et al. Clinical Characteristics of Coronavirus Disease 2019 in China. N Engl J Med 2020.
- Tian S, Hu N, Lou J, et al. Characteristics of COVID-19 infection in Beijing. J Infect 2020; 80(4): 401-6.
- Wang D, Hu B, Hu C, et al. Clinical Characteristics of 138 Hospitalized Patients With 2019 Novel Coronavirus-Infected Pneumonia in Wuhan, China. JAMA 2020.
- Xu YH, Dong JH, An WM, et al. Clinical and computed tomographic imaging features of novel coronavirus pneumonia caused by SARS-CoV-2. J Infect 2020; 80(4): 394-400.
- Zhang JJ, Dong X, Cao YY, et al. Clinical characteristics of 140 patients infected with SARS-CoV-2 in Wuhan, China. Allergy 2020.
- Chen T, Wu D, Chen H, et al. Clinical characteristics of 113 deceased patients with coronavirus disease 2019: retrospective study. BMJ 2020; 368: m1091.
- Wu Z, McGoogan JM. Characteristics of and Important Lessons From the Coronavirus Disease 2019 (COVID-19) Outbreak in China: Summary of a Report of 72314 Cases From the Chinese Center for Disease Control and Prevention. JAMA 2020.
- Wu C, Chen X, Cai Y, et al. Risk Factors Associated With Acute Respiratory Distress Syndrome and Death in Patients With Coronavirus Disease 2019 Pneumonia in Wuhan, China. JAMA Intern Med 2020.
- Barnett K, Mercer SW, Norbury M, Watt G, Wyke S, Guthrie B. Epidemiology of multimorbidity and implications for health care, research, and medical education: a cross-sectional study. Lancet 2012; 380(9836): 37-43.
- Zhou F, Yu T, Du R, et al. Clinical course and risk factors for mortality of adult inpatients with COVID-19 in Wuhan, China: a retrospective cohort study. Lancet 2020; 395(10229): 1054-62.
- Collins GS, Reitsma JB, Altman DG, Moons KG. Transparent reporting of a multivariable prediction model for individual prognosis or diagnosis (TRIPOD): the TRIPOD statement. BMJ 2015; 350: g7594.
- Richardson S, Hirsch JS, Narasimhan M, et al. Presenting Characteristics, Comorbidities, and Outcomes Among 5700 Patients Hospitalized With COVID-19 in the New York City Area. JAMA 2020.
- Barnes BJ, Adrover JM, Baxter-Stoltzfus A, et al. Targeting potential drivers of COVID-19: Neutrophil extracellular traps. J Exp Med 2020; 217(6).
- Tan L, Wang Q, Zhang D, et al. Lymphopenia predicts disease severity of COVID-19: a descriptive and predictive study. Signal Transduct Target Ther 2020; 5: 33.
- Stebbing J, Phelan A, Griffin I, et al. COVID-19: combining antiviral and anti-inflammatory treatments. Lancet Infect Dis 2020; 20(4): 400-2.
Table 1: Characteristics of the derivation and external validation cohorts
Wuhan cohort (n=775) | KCH cohort (n=226) | |||
---|---|---|---|---|
Demographic | No. (%) | No. (%) | ||
Age (median (IQR)) | 775 | 61 (50-68) | 226 | 74 (59-85) |
Sex (male) | 775 | 379 (48·9%) | 226 | 124 (54·9%) |
Outcome | ||||
Death | 773 | 33 (4·3%) | 226 | 77 (34·1%) |
Poor outcome | 772 | 75 (9·7%) | 226 | 97 (42·9%) |
Premorbid Conditions | ||||
Chronic lung disease | 771 | 48 (6·2%) | ·· | ·· |
Diabetes Mellitus | 771 | 106 (13·7%) | ·· | ·· |
Immunocompromised | 771 | 12 (1·5%) | ·· | ·· |
Malignancy | 771 | 24 (3·1%) | ·· | ·· |
Hypertension | 771 | 239 (30·8%) | ·· | ·· |
Heart disease | 771 | 85 (11·0%) | ·· | ·· |
Chronic renal disease | 771 | 25 (3·2%) | ·· | ·· |
Symptoms | ||||
Fever | 773 | 532 (68·6%) | ·· | ·· |
Cough | 773 | 528 (68·1%) | ·· | ·· |
Fatigue | 773 | 421 (54·3%) | ·· | ·· |
Dyspnoea | 773 | 355 (45·8%) | ·· | ·· |
Diarrhoea | 773 | 36 (4·6%) | ·· | ·· |
Laboratory tests (median (IQR)) | ||||
Neutrophil count (10^9/L) | 739 | 3·5 (2·7-4·9) | 226 | 4·9 (3·4-6·9) |
Lymphocyte (10^9/L) | 743 | 1·4 (1·1-1·9) | 226 | 1·1 (0·7-1·6) |
Platelet (10^9/L) | 732 | 224 (180-274) | 226 | 211 (160-284) |
C-reactive protein (mg/L) | 684 | 2·7 (0·9-14·1) | 226 | 56·0 (21·1-115·5) |
D-dimer (mg/L) | 581 | 0·4 (0·2-1·1) | ·· | ·· |
Creatinine (μmol/L) | 728 | 63·9 (54·1-76·4) | 226 | 90·0 (68·0-126·0) |
Alanine aminotransferase (IU/L) | 723 | 22·9 (14·8-39·5) | ·· | ·· |
Data are median (IQR) or number (%). Two patients had missing data for death and three patients had missing data for poor outcome.
Table 2: Multivariable logistic regression analysis of risk factors associated with Death and Poor outcome in COVID-19 patients in the derivation cohort
Death Odds Ratio† (95%CI) | Poor outcome Odds Ratio (95%CI) |
|||||
---|---|---|---|---|---|---|
Predictor | DCS* 30/769 (3·9%) | DCSL 19/651 (2·9%) | DL 20/653 (3·1%) | DCS 72/768 (9·4%) | DCSL 57/651 (8·8%) | DL 58/653 (8·9%) |
Demographic | ||||||
Age | 1·05 (1·01-1·08) | 1·00 (0·95-1·05) | 1·01 (0·96-1·07) | 1·04 (1·02-1·07) | 1·02 (0·99-1·05) | 1·03 (1·000-1·058) |
Sex (male) | 1·37 (0·62-3·03) | 0·42 (0·12-1·49) | 0·45 (0·13-1·50) | 1·23 (0·72-2·09) | 0·60 (0·28-1·29) | 0·62 (0·30-1·28) |
Premorbid Conditions | ||||||
Chronic lung disease | 3·00 (1·07-8·37) | 1·50 (0·26-8·52) | ·· | 3·42 (1·61-7·25) | 2·63 (0·81-8·51) | ·· |
Diabetes Mellitus | 1·56 (0·60-4·09) | 1·37 (0·35-5·38) | ·· | 1·00 (0·50-2·01) | 0·75 (0·28-2·01) | ·· |
Immunocompromised | 3·97 (0·64-24·65) | ·· | ·· | 4·19 (1·05-16·75) | 4·82 (0·63-36·76) | ·· |
Malignancy | 1·02 (0·15-7·05) | 1·00 (0·13-7·73) | ·· | 1·00 (0·25-3·99) | 0·49 (0·08-2·88) | ·· |
Hypertension | 1·41 (0·62-3·23) | ·· | ·· | 1·94 (1·11-3·39) | 1·62 (0·73-3·60) | ·· |
Heart disease | 1·97 (0·77-5·02) | ·· | ·· | 2·10 (1·08-4·08) | 2·09 (0·80-5·43) | ·· |
Chronic renal disease | 3·75 (0·92-15·30) | ·· | ·· | 2·70 (0·90-8·06) | 0·70 (0·11-4·62) | ·· |
Symptoms | ||||||
Fever | ·· | ·· | ·· | 1·03 (0·57-1·85) | 1·18 (0·53-2·66) | ·· |
Cough | 2·10 (0·70-6·32) | 2·57 (0·53-12·47) | ·· | 1·00 (0·53-1·88) | 0·55 (0·25-1·22) | ·· |
Fatigue | ·· | ·· | ·· | 1·10 (0·62-1·96) | 1·22 (0·56-2·66) | ·· |
Dyspnoea | 4·59 (1·79-11·79) | 2·35 (0·65-8·47) | ·· | 2·97 (1·64-5·39) | 3·47 (1·55-7·75) | ·· |
Diarrhoea | 1·00 (0·11-8·94) | ·· | ·· | 0·81 (0·17-3·78) | 0·07 (0·00-19·37) | ·· |
Laboratory tests | ||||||
Neutrophil count (10^9/L) | ·· | 1·20 (1·03-1·39) | 1·25 (1·09-1·44) | ·· | 1·28 (1·12-1·47) | 1·29 (1·14-1·46) |
Lymphocyte count (10^9/L) | ·· | 0·24 (0·05-1·04) | 0·27 (0·06-1·15) | ·· | 0·29 (0·12-0·72) | 0·31 (0·14-0·68) |
Platelet count (10^9/L) | ·· | 0·99 (0·987-1·001) | 0·99 (0·988-1·002) | ·· | 1·00 (0·992-1·001) | 1·00 (0·993-1·001) |
C-reactive protein (mg/L) | ·· | 1·01 (1·003-1·025) | 1·01 (1·004-1·024) | ·· | 1·01 (0.998-1·018) | 1·01 (1.000-1·017) |
Creatinine (μmol/L) | ·· | 1·00 (0.998-1·005) | 1·00 (0.998-1·005) | ·· | 1·01 (1·005-1·021) | 1·01 (1·003-1·016) |
* Initials specifying predictor categories examined in each model: D – Demographic, C – Premorbid Conditions, S – Symptoms, L – Laboratory Results. Data in the table head are number with outcome/number with complete data included in model (% with outcome).Two patients had missing data for death and three patients had missing data for poor outcome.
† For continuous value (age and laboratory tests), the OR interpretation is that the odds of having the outcome increase a value of OR-1 for a one-unit increase in the value. For example, for Death DCS model, the OR for Death is 1·06. This means that every 1 year increase in age is associated with a 6% increase in the odds of death (e.g. across the 18 year interquartile range of age, this equates to an OR of 2·85).
·· indicates the corresponding predictor is not included in the model
Table 3: Internally and externally validated performances of prediction models: discrimination, calibration and clinical usefulness
Internal cross-validation | Externally validation | |||||||
---|---|---|---|---|---|---|---|---|
Death | Poor outcome | Death | Poor outcome | |||||
DCS[a] N: 769 (3·9%) | DCSL[a] N: 651 (2·9%) | DL[a] N: 653 (3·1%) | DCS[a] N: 768 (9·4%) | DCSL[a] N: 651 (8·8%) | DL[a] N: 653 (8·9%) | DL[a] N: 226 (34·1%) | DL[a] N: 226 (42·9%) |
|
Discrimination and calibration | ||||||||
C-index | 0·79 | 0·89 | 0·91 | 0·80 | 0·88 | 0·88 | 0·74 | 0·72 |
Calibration in the large (95% CI)[b] | 0·384 (-0·077-0·846) | 0·316 (-0·078-0·711) | 0·189 (-0·104-0·481) | 0·132 (-0·133-0·397) | 0·059 (-0·169-0·287) | 0·008 (-0·131-0·147) | 0·251 (0·051-0·451) | 0·276 (0·086-0·466) |
Calibration slope (95% CI)[b] | 0·287 (-0·544-1·117) | 0·460 (-0·288-1·208) | 0·981 (0·244-1·718) | 0·855 (0·340-1·370) | 0·894 (0·507-1·281) | 1·036 (0·784-1·288) | 0·543 (0·202-0·885) | 0·540 (0·212-0·868) |
Clinical Usefulness[c] | ||||||||
Positive predictive value | 0·01 | 0·42 | 0·52 | 0·53 | 0·64 | 0·72 | 0·69 | 0·67 |
Negative predictive value | 0·96 | 0·98 | 0·98 | 0·91 | 0·94 | 0·94 | 0·71 | 0·65 |
Sensitivity | 0·003 | 0·25 | 0·33 | 0·09 | 0·37 | 0·36 | 0·23 | 0·40 |
Specificity | 0·997 | 0·99 | 0·99 | 0·99 | 0·98 | 0·99 | 0·95 | 0·85 |
Clinical Usefulness[d] Low risk vs high/very high | ||||||||
Positive predictive value | 0·07 | 0·25 | 0·25 | 0·19 | 0·22 | 0·17 | 0·55 | 0·44 |
Negative predictive value | 0·997 | 0·998 | 0·997 | 0·99 | 0·99 | 0·99 | 0·85 | 0·74 |
Sensitivity | 0·97 | 0·95 | 0·90 | 0·96 | 0·95 | 0·97 | 0·77 | 0·95 |
Specificity | 0·51 | 0·92 | 0·91 | 0·57 | 0·67 | 0·54 | 0·68 | 0·11 |
Clinical Usefulness[d] Low/high risk vs very high | ||||||||
Positive predictive value | 0·32 | 0·32 | 0·28 | 0·67 | 0·60 | 0·60 | 0·58 | 0·72 |
Negative predictive value | 0·97 | 0·995 | 0·99 | 0·93 | 0·96 | 0·96 | 0·78 | 0·65 |
Sensitivity | 0·30 | 0·84 | 0·75 | 0·25 | 0·53 | 0·53 | 0·58 | 0·61 |
Specificity | 0·97 | 0·95 | 0·94 | 0·99 | 0·97 | 0·96 | 0·78 | 0·75 |
[a] Initials specifying predictor categories examined in each model: D – Demographic, C – Premorbid Conditions, S – Symptoms, L – Laboratory Results. Data in the table head are number with complete data included in model (% with outcome).Two patients had missing data for death and three patients had missing data for poor outcome.
[b] Calibration was examined using quintiles of predicted risk. Good calibration is demonstrated by calibration-in-the-large close to zero, and calibration slope close to one.
[c] Metrics reported on default probability cut-off of 0·5.
[d] Metrics reported using selected probability cut offs for low risk vs high or very high (0·039 for death, 0·030 for poor outcome), and for low or high risk vs very high (0·069 for death, 0·265 for poor outcome). See Table S3 for details.